Short-term traffic flow prediction method and system based on convolutional neural network
A convolutional neural network and traffic flow technology, applied in short-term traffic flow prediction methods and systems, can solve problems such as high prediction error, inability to obtain, and inability to judge the strength of influence between intersections, so as to achieve accurate prediction and reduce adverse effects Effect
- Summary
- Abstract
- Description
- Claims
- Application Information
AI Technical Summary
Problems solved by technology
Method used
Image
Examples
Embodiment Construction
[0086] The following will clearly and completely describe the technical solutions in the embodiments of the present invention with reference to the accompanying drawings in the embodiments of the present invention. Obviously, the described embodiments are only some, not all, embodiments of the present invention. Based on the embodiments of the present invention, all other embodiments obtained by persons of ordinary skill in the art without creative efforts fall within the protection scope of the present invention. The present invention can use the deep learning method to more effectively utilize the characteristics of the current large amount of data to train and obtain a more accurate model.
[0087] The terms involved in the present invention are explained as follows:
[0088] (1) traffic flow data set, the elements contained in it are traffic flow data; wherein, traffic flow data: for each intersection with monitoring, the number of vehicles passing through in a certain per...
PUM
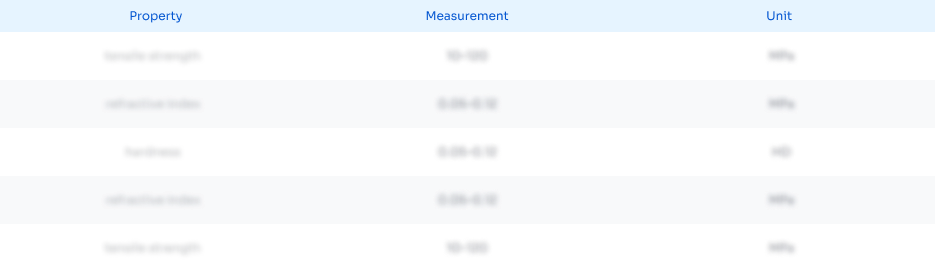
Abstract
Description
Claims
Application Information
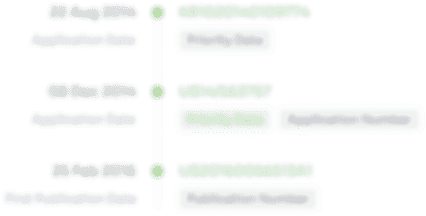
- R&D Engineer
- R&D Manager
- IP Professional
- Industry Leading Data Capabilities
- Powerful AI technology
- Patent DNA Extraction
Browse by: Latest US Patents, China's latest patents, Technical Efficacy Thesaurus, Application Domain, Technology Topic, Popular Technical Reports.
© 2024 PatSnap. All rights reserved.Legal|Privacy policy|Modern Slavery Act Transparency Statement|Sitemap|About US| Contact US: help@patsnap.com