Deep learning Residue2vec-based protein structure prediction method
A protein structure and prediction method technology, which is applied in the field of protein structure prediction based on deep learning Residue2vec, can solve the problems of poor conformation space sampling ability and insufficient conformation update accuracy, and achieve high matching degree and high prediction accuracy.
- Summary
- Abstract
- Description
- Claims
- Application Information
AI Technical Summary
Problems solved by technology
Method used
Image
Examples
Embodiment Construction
[0029] The present invention will be further described below in conjunction with the accompanying drawings.
[0030] refer to figure 1 and figure 2 , a protein structure prediction method based on deep learning Residue2vec, the conformational space optimization method includes the following steps:
[0031] 1) given input sequence information;
[0032] 2) Build the residue vector in the template library:
[0033] 2.1) Download the resolution less than from the protein database (PDB) website high-precision protein, where is the distance unit, m; removing redundant polypeptide chains whose similarity is greater than a preset threshold (for example, 30%), to obtain a non-redundant protein template library;
[0034] 2.2) Segment the non-redundant protein template into residues of length n by a sliding window;
[0035] 2.3) Through the CBOW model combined with Huffman coding, the residue model is modeled in the neural network, and the representation of the residue in the ...
PUM
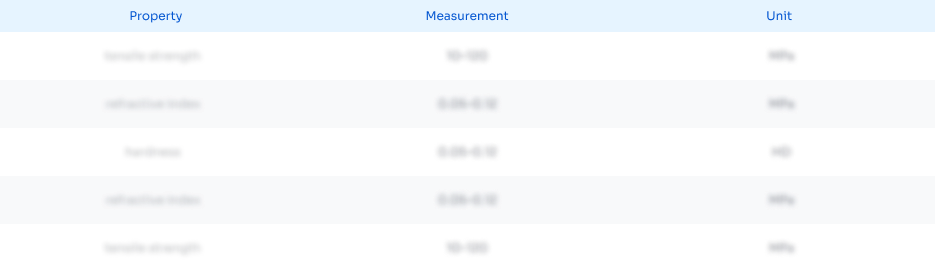
Abstract
Description
Claims
Application Information
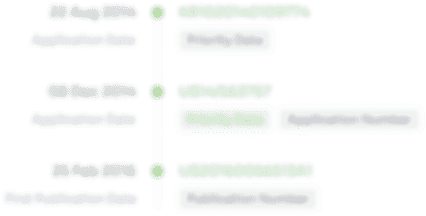
- Generate Ideas
- Intellectual Property
- Life Sciences
- Materials
- Tech Scout
- Unparalleled Data Quality
- Higher Quality Content
- 60% Fewer Hallucinations
Browse by: Latest US Patents, China's latest patents, Technical Efficacy Thesaurus, Application Domain, Technology Topic, Popular Technical Reports.
© 2025 PatSnap. All rights reserved.Legal|Privacy policy|Modern Slavery Act Transparency Statement|Sitemap|About US| Contact US: help@patsnap.com