Voice identification method using long-short term memory model recurrent neural network
A technology of recurrent neural network and long-term short-term memory, which is applied in speech recognition, speech analysis, instruments, etc., and can solve problems such as inconsistency in training goals
- Summary
- Abstract
- Description
- Claims
- Application Information
AI Technical Summary
Problems solved by technology
Method used
Image
Examples
Embodiment Construction
[0058] It should be noted that, in the case of no conflict, the embodiments in the present application and the features in the embodiments can be combined with each other. The present invention will be further described in detail below in conjunction with the drawings and specific embodiments.
[0059] figure 1 It is a flowchart of the training process of the present invention, including voice data and text data, acoustic model and language model, RNN sensor, decoding, and model parameters.
[0060] Speech data and text data are for training on speech data and text data.
[0061] The acoustic model and the language model are to process speech data and text data using the acoustic model and the language model.
[0062] The RNN sensor predicts the correspondence between each phoneme and the previous phoneme, thereby producing a jointly trained acoustic and language model. The RNN sensor determines a separate distribution Pr(k| t, u), for a length U and the target sequence z, t...
PUM
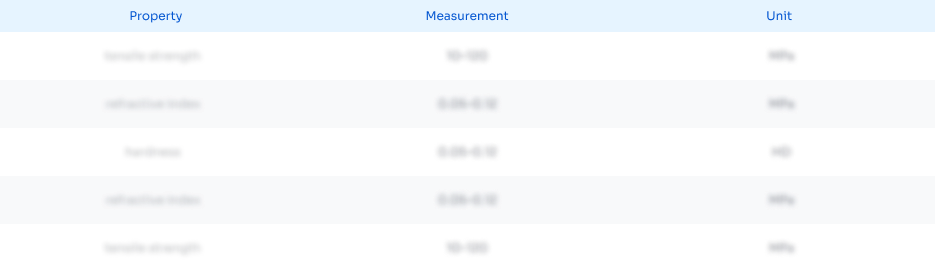
Abstract
Description
Claims
Application Information
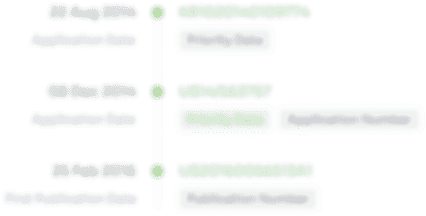
- R&D Engineer
- R&D Manager
- IP Professional
- Industry Leading Data Capabilities
- Powerful AI technology
- Patent DNA Extraction
Browse by: Latest US Patents, China's latest patents, Technical Efficacy Thesaurus, Application Domain, Technology Topic, Popular Technical Reports.
© 2024 PatSnap. All rights reserved.Legal|Privacy policy|Modern Slavery Act Transparency Statement|Sitemap|About US| Contact US: help@patsnap.com