Method for applying multi-criterion fusion to feature selection of high-dimensional small sample data
A technology that integrates application and feature selection. It is used in instruments, character and pattern recognition, computer components, etc., and can solve problems such as poor algorithm stability.
- Summary
- Abstract
- Description
- Claims
- Application Information
AI Technical Summary
Problems solved by technology
Method used
Image
Examples
Embodiment
[0022] Embodiment: the method for applying multi-criteria fusion to high-dimensional small sample data feature selection includes the following steps:
[0023] Step 1): The specific method of clustering in step 1) is to cluster the training samples by using the k-means clustering method, wherein the kth clustering uses the set G k to represent, assuming G k Contains n data{x 1 ,x 2 ,...x n}, the task of k-means clustering is to find a set of m representative points Y={y 1 ,y 2 ,...y m} so that the objective function The smaller the better, where y k yes k The cluster center and the number of clusters are determined by experiments. The value of k described in this embodiment is 8.
[0024] Step 2): Use the Fisher Ratio method and the ReliefF method to perform feature selection on the clustered samples respectively;
[0025] Step 3): Fusion feature selection results and different penalty factors are added to each class, and then the fusion result is used to train the...
PUM
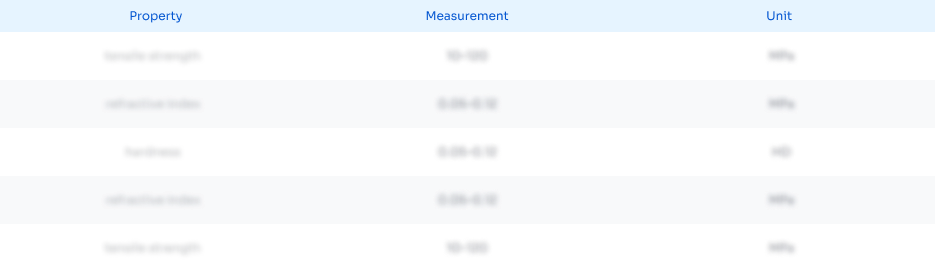
Abstract
Description
Claims
Application Information
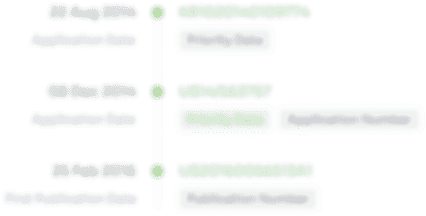
- R&D Engineer
- R&D Manager
- IP Professional
- Industry Leading Data Capabilities
- Powerful AI technology
- Patent DNA Extraction
Browse by: Latest US Patents, China's latest patents, Technical Efficacy Thesaurus, Application Domain, Technology Topic, Popular Technical Reports.
© 2024 PatSnap. All rights reserved.Legal|Privacy policy|Modern Slavery Act Transparency Statement|Sitemap|About US| Contact US: help@patsnap.com