Photovoltaic string fault diagnosis method based on kernel function limit learning machine
An extreme learning machine and fault diagnosis technology, applied in the monitoring of photovoltaic modules, photovoltaic power generation, photovoltaic systems, etc., can solve the problems of fault diagnosis and classification of photovoltaic power generation strings that have not yet been seen, and achieve shortened training time, high sensitivity, The effect of training accuracy improvement
- Summary
- Abstract
- Description
- Claims
- Application Information
AI Technical Summary
Problems solved by technology
Method used
Image
Examples
Embodiment Construction
[0022] The technical solution of the present invention will be specifically described below in conjunction with the accompanying drawings.
[0023] The present invention provides a method for fault diagnosis and classification of photovoltaic power generation strings based on kernel function extreme learning machine. The flow chart is as follows figure 1 shown. figure 2 It is the topological diagram of the photovoltaic power generation system in this embodiment. The system is composed of S×P solar modules. By simulating different fault conditions of photovoltaic power generation strings, such as open circuit, short circuit, partial shadow and other working states, the system can be used under different climate conditions. Next, select a different time period to obtain a large number of internal equivalent parameters for each fault situation, which specifically includes the following steps:
[0024] Step S1: Scan the volt-ampere characteristics of the photovoltaic power gener...
PUM
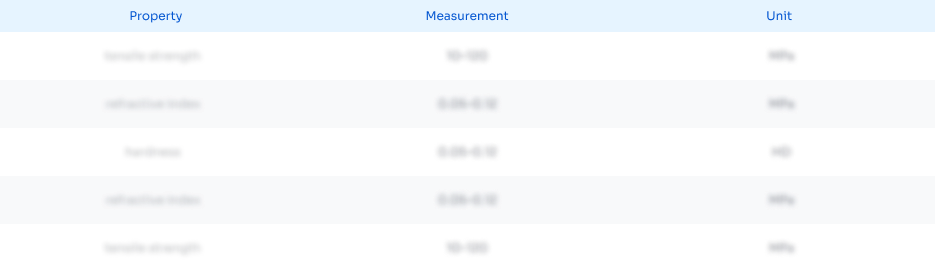
Abstract
Description
Claims
Application Information
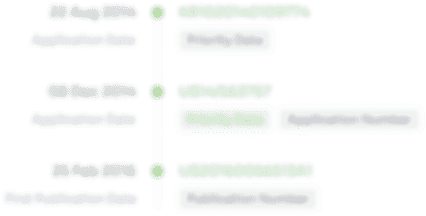
- R&D Engineer
- R&D Manager
- IP Professional
- Industry Leading Data Capabilities
- Powerful AI technology
- Patent DNA Extraction
Browse by: Latest US Patents, China's latest patents, Technical Efficacy Thesaurus, Application Domain, Technology Topic, Popular Technical Reports.
© 2024 PatSnap. All rights reserved.Legal|Privacy policy|Modern Slavery Act Transparency Statement|Sitemap|About US| Contact US: help@patsnap.com