Target tracking method and system based on partitioned multi-example learning algorithm
A multi-instance learning and target tracking technology, which is applied in the field of target tracking methods and systems based on the block multi-instance learning algorithm, can solve the problems of tracking result drift, target tracking performance is not high enough, and MIL algorithm calculation is time-consuming. The effect of stable target tracking and improved performance
- Summary
- Abstract
- Description
- Claims
- Application Information
AI Technical Summary
Problems solved by technology
Method used
Image
Examples
Embodiment Construction
[0036] The present invention will be further described in detail below in conjunction with the accompanying drawings and specific embodiments.
[0037] The basic idea of existing multi-instance learning algorithms is to implement multi-instance learning algorithms under the framework of Online Boosting. The algorithm collects examples in the target and background areas respectively to form marked packets as training samples, namely: {(X 1 ,y 1 ),…,(X i ,y i ),…,(X m ,y m )}. Among them, X i ={x i1 ,x i2 ,,x in} for example {x i1 ,x i2 ,,x in} consisting of packets, y i mark for the package. when y i = 1, the package is a positive package, when y i =0, the packet is a negative packet.
[0038] The positive packet consists of samples collected within the domain of the target location in the current frame: in is the current position of the target, and r is the radius of the circle where the sample is collected. Collect examples at the background to form a ...
PUM
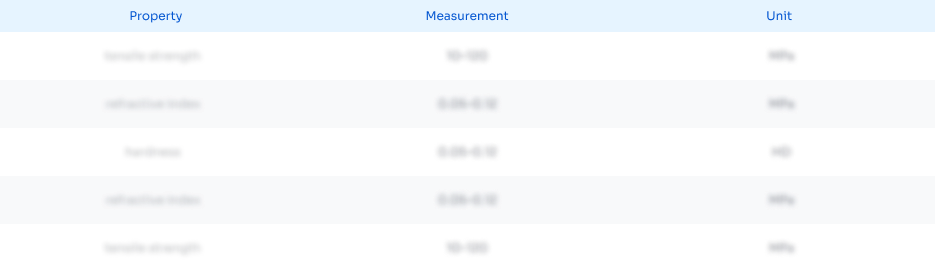
Abstract
Description
Claims
Application Information
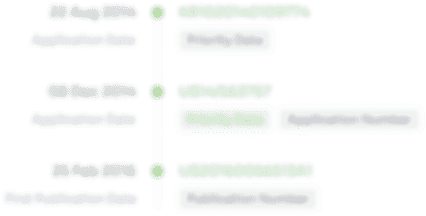
- R&D Engineer
- R&D Manager
- IP Professional
- Industry Leading Data Capabilities
- Powerful AI technology
- Patent DNA Extraction
Browse by: Latest US Patents, China's latest patents, Technical Efficacy Thesaurus, Application Domain, Technology Topic, Popular Technical Reports.
© 2024 PatSnap. All rights reserved.Legal|Privacy policy|Modern Slavery Act Transparency Statement|Sitemap|About US| Contact US: help@patsnap.com