Wavelet packet frequency domain signal manifold studying failure diagnosis method
A manifold learning and frequency domain signal technology, applied in the field of wavelet packet frequency domain signal manifold learning fault diagnosis, can solve the problems of nonlinear data crowding, low-dimensional manifold expression is not clear enough, etc., and achieve the effect of reducing data length
- Summary
- Abstract
- Description
- Claims
- Application Information
AI Technical Summary
Problems solved by technology
Method used
Image
Examples
Embodiment Construction
[0019] The present invention will be described in detail below in conjunction with the accompanying drawings and embodiments.
[0020] Since the vibration signal of the machine equipment is the main signal used for diagnosing and analyzing the operating state of the equipment, a lot of characteristic information contained in the vibration signal is related to the operating state of the machine, but it is difficult to effectively judge by extracting a characteristic information of the vibration signal alone The operating state of the device. Such as figure 1 As shown, the present invention provides a wavelet packet frequency domain signal manifold learning fault diagnosis method, the method is to decompose the vibration data obtained by specific parts of the machine equipment through wavelet packet decomposition to generate multi-layer detail data, which is composed of these layered data High-dimensional data vectors, different layers of data contain different characteristic i...
PUM
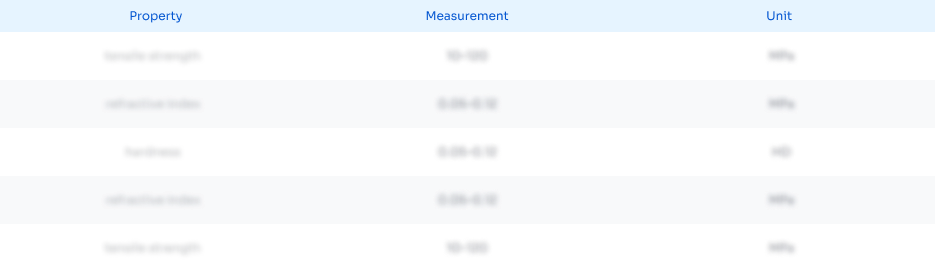
Abstract
Description
Claims
Application Information
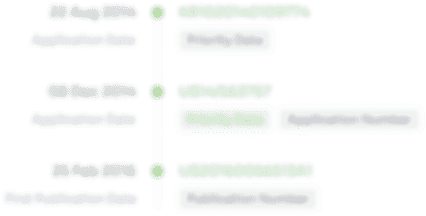
- R&D Engineer
- R&D Manager
- IP Professional
- Industry Leading Data Capabilities
- Powerful AI technology
- Patent DNA Extraction
Browse by: Latest US Patents, China's latest patents, Technical Efficacy Thesaurus, Application Domain, Technology Topic, Popular Technical Reports.
© 2024 PatSnap. All rights reserved.Legal|Privacy policy|Modern Slavery Act Transparency Statement|Sitemap|About US| Contact US: help@patsnap.com