Network traffic abnormality detection method based on SVM (Support Vector Machine)
A network traffic and anomaly detection technology, applied in the field of network security, can solve problems such as large dimension and hacker attack
- Summary
- Abstract
- Description
- Claims
- Application Information
AI Technical Summary
Problems solved by technology
Method used
Image
Examples
Embodiment Construction
[0054] In order to further understand the technical content of the present invention, the present invention will be further described below in conjunction with the accompanying drawings.
[0055] Such as figure 1 As shown, it is a flow chart of a method for detecting network traffic anomalies based on SVM provided by the present invention, and the method includes the following steps:
[0056] Step S1, reading historical network traffic data.
[0057] Step S2, extracting network traffic characteristics of the historical network traffic data.
[0058] Network traffic features are mainly statistical features, including the attributes of packets and flows. These statistical features are represented by feature vectors, such as a piece of network flow data X. The feature description based on this flow can be expressed as X=(x 1 ,x 2 ,...,x n ), where x i represents the i-th feature.
[0059] Step S3, performing data standardization on the network traffic characteristics. It i...
PUM
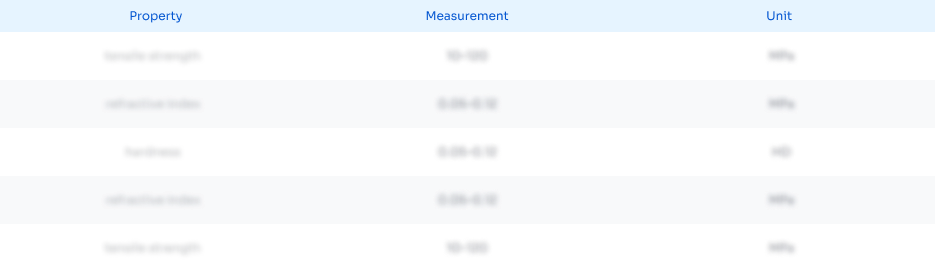
Abstract
Description
Claims
Application Information
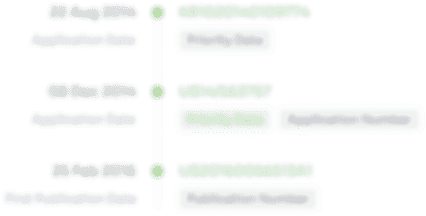
- R&D Engineer
- R&D Manager
- IP Professional
- Industry Leading Data Capabilities
- Powerful AI technology
- Patent DNA Extraction
Browse by: Latest US Patents, China's latest patents, Technical Efficacy Thesaurus, Application Domain, Technology Topic, Popular Technical Reports.
© 2024 PatSnap. All rights reserved.Legal|Privacy policy|Modern Slavery Act Transparency Statement|Sitemap|About US| Contact US: help@patsnap.com