Clustering model based high-dimensional data stream outlier detection method
A technology for high-dimensional data and data points, applied in structured data retrieval, database models, relational databases, etc., can solve problems such as low processing efficiency and meaninglessness, and achieve improved processing speed, reduced errors, and outlier detection good accuracy
- Summary
- Abstract
- Description
- Claims
- Application Information
AI Technical Summary
Problems solved by technology
Method used
Image
Examples
Embodiment Construction
[0028] The method of the present invention will be further described in detail below with reference to the accompanying drawings and specific embodiments. It should be understood that these examples are only intended to illustrate the present invention and not to limit the scope of the present invention.
[0029] Some specific parameters are initialized before the algorithm is executed. The algorithm tolerates the maximum number of clusters K, the minimum distance threshold r1 between data points and clusters, and the minimum distance threshold r2 between clusters and clusters (mindist in step 2). When judging outlier clusters The minimum number of data points m in the cluster (N in step 3 min ) and the maximum time interval t (Tr in step 3), etc.
[0030] The concrete steps of the present invention include:
[0031] Step 1: As figure 1 As shown in the figure, data preprocessing is performed, the input training set is clustered, and then the feature dimension of each cluste...
PUM
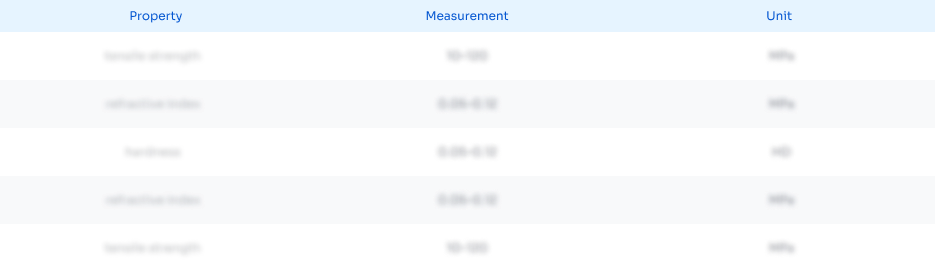
Abstract
Description
Claims
Application Information
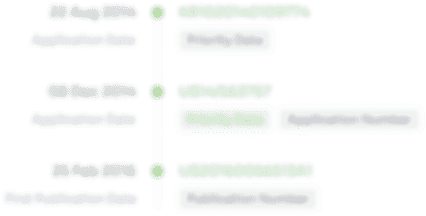
- R&D Engineer
- R&D Manager
- IP Professional
- Industry Leading Data Capabilities
- Powerful AI technology
- Patent DNA Extraction
Browse by: Latest US Patents, China's latest patents, Technical Efficacy Thesaurus, Application Domain, Technology Topic, Popular Technical Reports.
© 2024 PatSnap. All rights reserved.Legal|Privacy policy|Modern Slavery Act Transparency Statement|Sitemap|About US| Contact US: help@patsnap.com