Polarization SAR terrain classification method based on deep learning and distance metric learning
A metric learning and deep learning technology, applied in the field of image classification and image processing, can solve the problems of high time complexity, limited classification accuracy, slow processing speed, etc., to achieve low time complexity, overcome low classification accuracy, improve classification The effect of precision
- Summary
- Abstract
- Description
- Claims
- Application Information
AI Technical Summary
Problems solved by technology
Method used
Image
Examples
Embodiment Construction
[0041] The present invention will be further described below in conjunction with the accompanying drawings.
[0042] Refer to attached figure 1 , the concrete steps of the present invention are as follows:
[0043] Step 1, input the polarimetric SAR image to be classified.
[0044] Step 2, filtering.
[0045] Using the Lee filter method with a filter window size of 7×7, the polarimetric SAR image to be classified is filtered to remove coherent speckle noise, and the filtered polarimetric SAR image is obtained.
[0046] Step 3, feature extraction.
[0047] (1) Calculate two scattering parameters, scattering entropy and scattering angle;
[0048] In the first step, the scattering entropy of the polarimetric SAR image is calculated according to the following formula:
[0049] H = Σ i = 1 3 - P i log ...
PUM
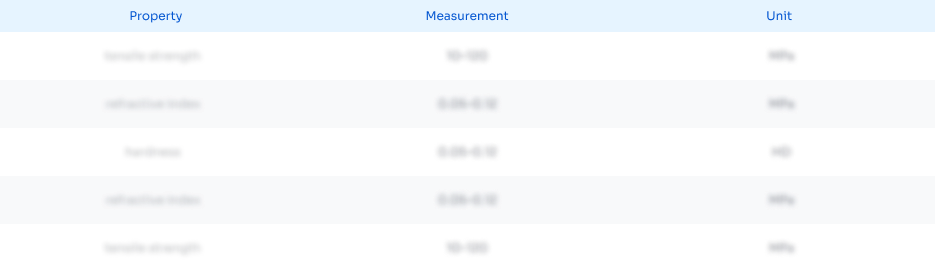
Abstract
Description
Claims
Application Information
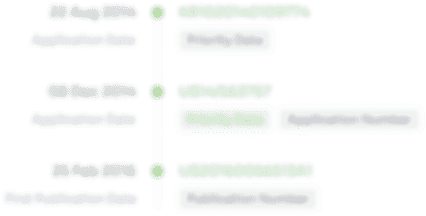
- R&D Engineer
- R&D Manager
- IP Professional
- Industry Leading Data Capabilities
- Powerful AI technology
- Patent DNA Extraction
Browse by: Latest US Patents, China's latest patents, Technical Efficacy Thesaurus, Application Domain, Technology Topic, Popular Technical Reports.
© 2024 PatSnap. All rights reserved.Legal|Privacy policy|Modern Slavery Act Transparency Statement|Sitemap|About US| Contact US: help@patsnap.com