A wrapper hyperspectral band selection method based on pixel clustering
A band selection and hyperspectral technology, which is applied in the field of image processing, can solve the problems of limited scope of use of supervised band selection methods, achieve the effects of optimizing clustering effects, improving band selection efficiency, and improving reliability
- Summary
- Abstract
- Description
- Claims
- Application Information
AI Technical Summary
Problems solved by technology
Method used
Image
Examples
Embodiment 1
[0026] The specific steps of the wrapper hyperspectral band selection method based on pixel clustering are as follows:
[0027] (1) Input the hyperspectral image to be selected by the band, assuming that the number of original bands of the image is P, convert the image into hyperspectral data in matrix form;
[0028] The hyperspectral image selected in this example is the Indiana hyperspectral image acquired by the airborne visible and infrared imaging spectrometer AVIRIS. The hyperspectral image contains 16 different types of ground objects, including soybeans, wheat, corn, Grassland, artificial buildings, roads, etc., these features are distributed in different areas of the map. The hyperspectral image contains 220 original bands, ie P=220, and the wavelength range is 0.4 μm-2.5 μm. The number of pixels in the hyperspectral image is 145*145, and the size of the hyperspectral image data converted into matrix form is 145*145*220. In order to facilitate subsequent data proces...
Embodiment 2
[0052] The wrapper formula hyperspectral band selection method based on pixel clustering is the same as in embodiment 1, wherein in step (3), a representative point is selected from the segmented superpixel block, and the specific steps include:
[0053] 3.1: In this example, 462 superpixel blocks are generated in step (2), assuming that there are j pixels in the i-th superpixel block, the spectral information of each point in the i-th superpixel block is recorded as where each element , k=1,2,...,j; both are a 220-dimensional feature vector, representing the spectral information of the point; then the average spectral value in the block is:
[0054]
[0055] 3.2: Calculate each pixel in the i-th superpixel block , k=1,2,...,j; and the average spectral value The correlation of j correlation values {corr 1 , corr 2 ,..., corr j};
[0056] 3.3: Select the pixel with the greatest correlation in each superpixel block as the most representative pixel point, and selec...
Embodiment 3
[0059] The wrapper type hyperspectral band selection method based on pixel clustering is the same as that in embodiment 1-2, wherein in step (4), the initial clustering results of 462 representative points are used as initial labels, and then support vector machine (SVM) is used to perform initial clustering Class results are optimized and classified to obtain the final clustering results of 462 representative points; the specific steps include:
[0060] 4.1: Perform k-medoids clustering on the obtained 462 representative pixel points, set 462 representative points to gather into 25 classes in this embodiment, then the initial clustering result of 462 representative points is expressed as {C k}, k=1,2,...,462; where C k ∈{1,2,...,25}; the specific steps of k-medoids clustering of 462 representative points include:
[0061] 4.1a: Enter the number of clustering categories. In this example, the number of clustering categories is 25, and 25 representative points are randomly sele...
PUM
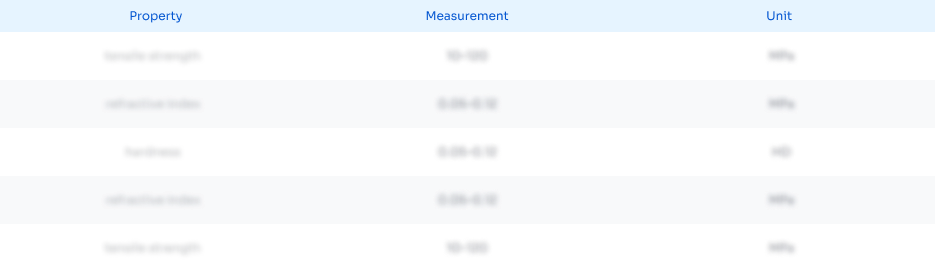
Abstract
Description
Claims
Application Information
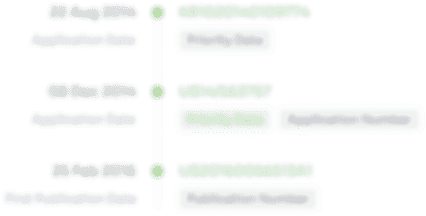
- R&D Engineer
- R&D Manager
- IP Professional
- Industry Leading Data Capabilities
- Powerful AI technology
- Patent DNA Extraction
Browse by: Latest US Patents, China's latest patents, Technical Efficacy Thesaurus, Application Domain, Technology Topic, Popular Technical Reports.
© 2024 PatSnap. All rights reserved.Legal|Privacy policy|Modern Slavery Act Transparency Statement|Sitemap|About US| Contact US: help@patsnap.com