Image pixel semantic annotation method with combination of multi-granularity context information
A technology of semantic annotation and image pixels, which is applied in the direction of instruments, character and pattern recognition, computer parts, etc., can solve the problems of identification and confusion, achieve high image annotation accuracy, reasonable design, and realize the effect of intelligence
- Summary
- Abstract
- Description
- Claims
- Application Information
AI Technical Summary
Problems solved by technology
Method used
Image
Examples
Embodiment Construction
[0032] Specific embodiments of the present invention will be described in detail below in conjunction with the accompanying drawings.
[0033] In the process of image understanding, context information plays an important role in different types of target recognition. The traditional second-order conditional random field model only describes local context information. In order to obtain global context information, the present invention uses the neighborhood base The clique is expanded into two grained context windows, the continuity of the semantic label categories at adjacent positions is considered in the fine-grained context window, and the homogeneous transfer probability of the semantic label is calculated by using the label smoothing parameters and the fine-grained context descriptor. In the granular context window, sparse representation is used to describe the co-occurrence of different types of semantic tags, and the spatial co-occurrence relationship of semantic categor...
PUM
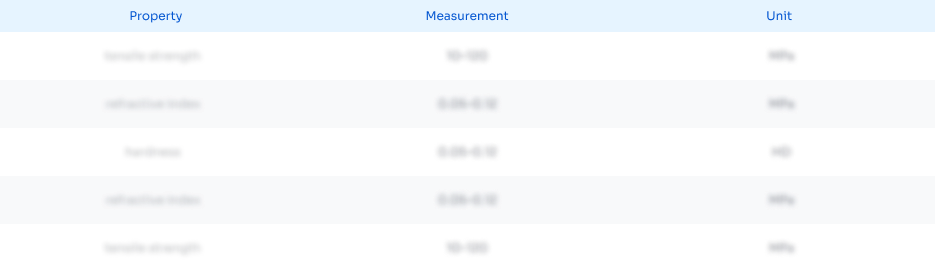
Abstract
Description
Claims
Application Information
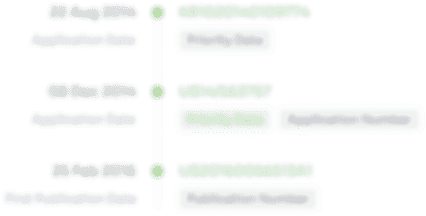
- R&D Engineer
- R&D Manager
- IP Professional
- Industry Leading Data Capabilities
- Powerful AI technology
- Patent DNA Extraction
Browse by: Latest US Patents, China's latest patents, Technical Efficacy Thesaurus, Application Domain, Technology Topic, Popular Technical Reports.
© 2024 PatSnap. All rights reserved.Legal|Privacy policy|Modern Slavery Act Transparency Statement|Sitemap|About US| Contact US: help@patsnap.com