Adaptive Unmixing Method for Hyperspectral Image Based on Region Segmentation
A hyperspectral image and region segmentation technology, which is applied in the field of image processing and can solve problems such as inapplicability to hyperspectral data
- Summary
- Abstract
- Description
- Claims
- Application Information
AI Technical Summary
Problems solved by technology
Method used
Image
Examples
Embodiment 1
[0048] The present invention is a hyperspectral image adaptive unmixing method based on region segmentation, referring to figure 1 , the specific implementation steps of the present invention include:
[0049] (1) Input hyperspectral image, hyperspectral image data Y∈R L×N where L represents the number of bands of hyperspectral data, N represents the total number of hyperspectral data samples, and R represents the real number domain; in this example, the rgb image of the 30th band of the input hyperspectral image is as follows figure 2 As shown, the figure contains three substances: vegetation, water and soil. Hyperspectral unmixing is to obtain the percentages of these three different substances, that is, the abundance map.
[0050] (2) Estimate hyperspectral data Y∈R using the minimum error hyperspectral signal recognition method L×N The signal subspace of the signal subspace, the dimension K of the signal subspace is obtained, that is, the number of endmembers of the hy...
Embodiment 2
[0060] The hyperspectral image adaptive unmixing method based on region segmentation is the same as embodiment 1, wherein step (5) described with L 1 / 2 Constrained non-negative matrix factorization method to obtain homogeneous regional data Y 1 The first-order abundance matrix X 1 , including the following steps:
[0061](5a) According to the hyperspectral imaging theory, the abundance matrix X of the data in the homogeneous region of the hyperspectral image 1 Add L to 1 / 2 Norm, get the sparse constraint expression as the abundance matrix X 1 The sparse constraint term, where x 1n (k) is the hyperspectral image homogeneous region data Y 1 The abundance of the kth endmember of the nth pixel in .
[0062] (5b) Add the sparse constraint item obtained in step (5a) to the objective function of the non-negative matrix factorization algorithm based on Euclidean distance , forming a new objective function:
[0063]
[0064] Condition: X 1 ≥0,1 T x 1 = 1 T
[0065] w...
Embodiment 3
[0072] The hyperspectral image adaptive unmixing method based on region segmentation is the same as embodiment 1-2, wherein step (6) uses L 1 / 2 -Semi_NMF method to get detail area data Y 2 The corresponding first-order abundance matrix X 2 and the second-order abundance matrix E, according to the following steps:
[0073] (6a) In hyperspectral image detail area Y 2 The bilinear model is used to express as follows
[0074] Y 2 =AX 2 +BE+M
[0075] in, Represents the first-order abundance matrix corresponding to the data in the bilinear region, where each column vector represents the abundance vector of the nth pixel, is a bilinear endmember matrix, Is the second-order abundance matrix corresponding to the data in the bilinear region, where each column vector represents the bilinear abundance vector of the nth pixel, Represents the noise matrix;
[0076] (6b) The abundance matrix X of the data in the homogeneous region of the hyperspectral image 2 Add L to 1 / ...
PUM
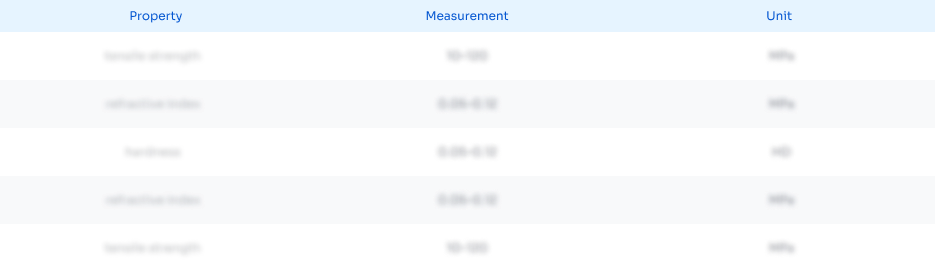
Abstract
Description
Claims
Application Information
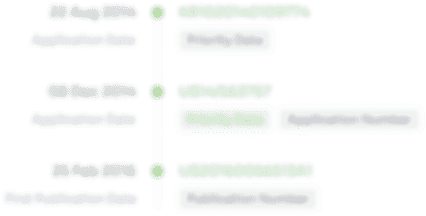
- R&D Engineer
- R&D Manager
- IP Professional
- Industry Leading Data Capabilities
- Powerful AI technology
- Patent DNA Extraction
Browse by: Latest US Patents, China's latest patents, Technical Efficacy Thesaurus, Application Domain, Technology Topic, Popular Technical Reports.
© 2024 PatSnap. All rights reserved.Legal|Privacy policy|Modern Slavery Act Transparency Statement|Sitemap|About US| Contact US: help@patsnap.com