Low-rank sparse neighborhood embedding method for super-resolution based on normlv features
A neighborhood embedding, low-resolution technology, applied in the field of image processing, which can solve the problems of non-one-to-one linear mapping, under-fitting, and algorithm time-consuming.
- Summary
- Abstract
- Description
- Claims
- Application Information
AI Technical Summary
Problems solved by technology
Method used
Image
Examples
Embodiment 1
[0064] The present invention is a low-rank sparse neighborhood embedding super-resolution method based on NormLV features, see figure 1 , the implementation steps are as follows:
[0065] Step 1: Read in a noise-free low-resolution color RGB image L t , the RGB image is converted into a YCbCr image in color space, the blue component Cb and the red component Cr are directly interpolated using the Bicubic interpolation method, and the brightness component Y is operated by the NormLV feature extraction method. In this example will Figure 7 (a) The test image used as the experiment.
[0066] Step 2: NormLV feature extraction: extract the NormLV feature from the brightness component Y of the low-resolution image to obtain the low-resolution image training set in is the i-th low-resolution image feature vector, and N is the total number of image blocks in the low-resolution image training set.
[0067] Step 3: Grouping: For low-resolution image training set X s Each vector ...
Embodiment 2
[0090] Low-rank sparse neighborhood embedding super-resolution method based on NormLV feature is the same as embodiment 1, see figure 1 , wherein the specific steps of the NormLV feature extraction method in step 2 are as follows:
[0091] 2a) Divide the brightness component Y of the low-resolution image from top to bottom and from left to right into image blocks with a size of s×s, and overlap 1 pixel between adjacent image blocks. In this example, figure 2 As the test image patch used in the experiment.
[0092] 2b) Extract the first-order gradient features of low-resolution image blocks▽ first gradient .
[0093] 2c) Extract Norm features of low-resolution image blocks▽ Norm, the so-called Norm feature is the value of the middle pixel minus the mean value of the block.
[0094] 2d) Extract LV features of low-resolution image blocks▽ LV , the LV feature is to combine the horizontal and vertical methods of the image, and subtract the pixel values in the four direction...
Embodiment 3
[0101] Low-rank sparse neighborhood embedding super-resolution method based on NormLV feature is the same as embodiment 1-2, see figure 1 , where the specific steps of the low-rank sparse neighborhood embedding algorithm in step 4 are as follows:
[0102] 4a) For each test image block In the low-resolution image training set X s find the most similar and get with related group G i , where G i Contains K+1 indexes;
[0103] 4b) will be compared with The associated K low-resolution image gradient feature vectors form a matrix in Similarly, the corresponding K high-resolution image intensity feature vectors form a matrix in
[0104] 4c) Input image block and low-resolution image feature matrix L i composition matrix
[0105] 4d) will and H i For low-rank matrix decomposition, the specific optimization function is:
[0106]
[0107]
[0108] in, and are low-resolution image feature matrix The low-rank component and sparse component of ; a...
PUM
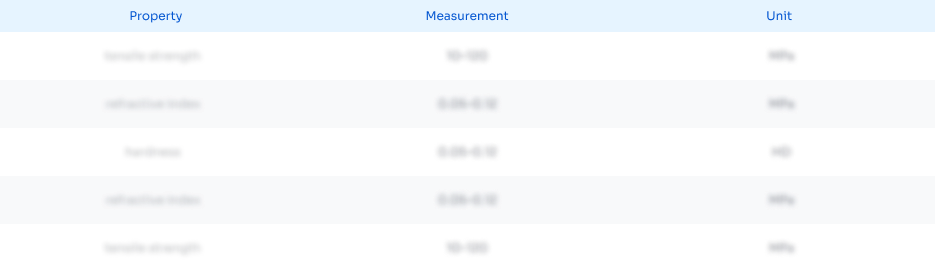
Abstract
Description
Claims
Application Information
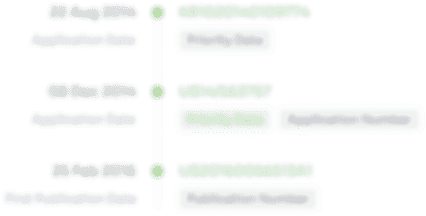
- R&D Engineer
- R&D Manager
- IP Professional
- Industry Leading Data Capabilities
- Powerful AI technology
- Patent DNA Extraction
Browse by: Latest US Patents, China's latest patents, Technical Efficacy Thesaurus, Application Domain, Technology Topic, Popular Technical Reports.
© 2024 PatSnap. All rights reserved.Legal|Privacy policy|Modern Slavery Act Transparency Statement|Sitemap|About US| Contact US: help@patsnap.com