Semi-supervision hyperspectral data dimension descending method based on largest neighbor boundary principle
A semi-supervised and nearest-neighbor technology, applied in the field of image processing, can solve the problems of poor universality, ignoring the spatial information of image data, and data without discriminative performance.
- Summary
- Abstract
- Description
- Claims
- Application Information
AI Technical Summary
Problems solved by technology
Method used
Image
Examples
Embodiment
[0046] 1b) In the training data set X, k samples are randomly selected for each class to form a supervised labeled sample set Y∈R D×Q , where Q=c×k, c is the number of categories, in the implementation example IndianPines data set of the present invention, c is 16, and k is {5,6,8};
[0047] 1c) In the labeled sample set Y, for each labeled sample y i Calculate its homogeneous neighbor set by Euclidean distance and a heterogeneous set of neighbors
[0048] Step 2: Generate the scatter matrix of the labeled sample set.
[0049] 2a) Generate the similarity scatter matrix of the labeled sample set by the similarity scatter matrix formula:
[0050] C = Σ i , j : y j ∈ N i o ...
PUM
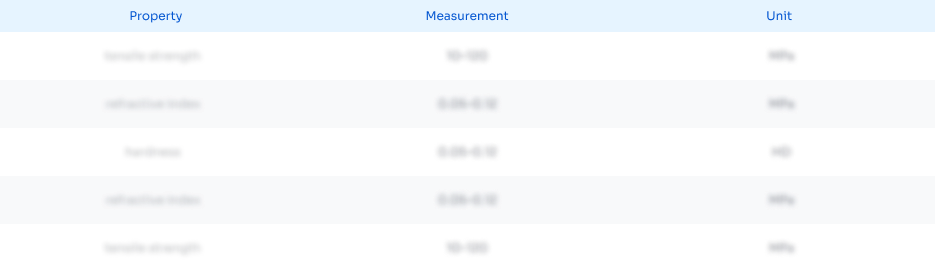
Abstract
Description
Claims
Application Information
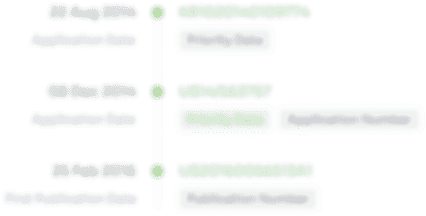
- R&D Engineer
- R&D Manager
- IP Professional
- Industry Leading Data Capabilities
- Powerful AI technology
- Patent DNA Extraction
Browse by: Latest US Patents, China's latest patents, Technical Efficacy Thesaurus, Application Domain, Technology Topic, Popular Technical Reports.
© 2024 PatSnap. All rights reserved.Legal|Privacy policy|Modern Slavery Act Transparency Statement|Sitemap|About US| Contact US: help@patsnap.com