Water quality parameter prediction method based on online sequential extreme learning machine
A technology of extreme learning machine and water quality parameters, which is applied in the fields of electrical digital data processing, special data processing applications, instruments, etc., and can solve problems such as restricting the popularization and application of artificial neural networks, complicated changing rules, and slow learning speed
- Summary
- Abstract
- Description
- Claims
- Application Information
AI Technical Summary
Problems solved by technology
Method used
Image
Examples
Embodiment Construction
[0025] Such as figure 1 As shown, a water quality parameter prediction method based on online sequential extreme learning machine, including the following steps:
[0026] Step 1: Collect the historical data of water quality parameters from the automatic water quality monitoring station and preprocess the data, and use the Newton interpolation method to complete the missing data in the historical data;
[0027] Step 2: Use the first 2 / 3 of the preprocessed historical data of water quality parameters as the learning sample set, and the last 1 / 3 of the data as the testing sample set;
[0028]Step 3: Take part of the data in the learning sample set to initialize the online sequential extreme learning machine OSELM, and then use the remaining data in the learning sample set to let the online sequential extreme learning machine OSELM learn. During the learning process, the remaining data in the learning sample set The water quality parameter values of the first several consecutiv...
PUM
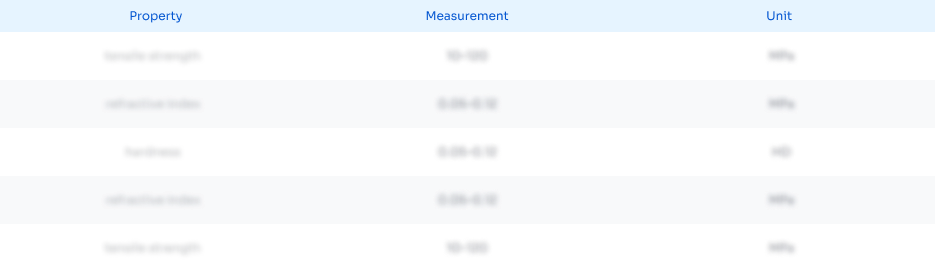
Abstract
Description
Claims
Application Information
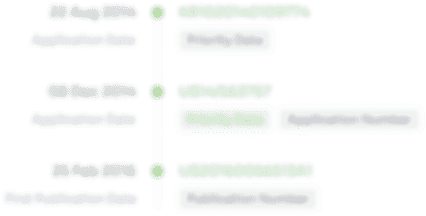
- Generate Ideas
- Intellectual Property
- Life Sciences
- Materials
- Tech Scout
- Unparalleled Data Quality
- Higher Quality Content
- 60% Fewer Hallucinations
Browse by: Latest US Patents, China's latest patents, Technical Efficacy Thesaurus, Application Domain, Technology Topic, Popular Technical Reports.
© 2025 PatSnap. All rights reserved.Legal|Privacy policy|Modern Slavery Act Transparency Statement|Sitemap|About US| Contact US: help@patsnap.com