Learning and anomaly detection method based on multi-feature motion modes of vehicle traces
A pattern learning, multi-feature technology, applied in two-dimensional position/channel control and other directions, can solve the problem of not considering abnormal direction, difficult to identify and so on
- Summary
- Abstract
- Description
- Claims
- Application Information
AI Technical Summary
Problems solved by technology
Method used
Image
Examples
Embodiment Construction
[0084] 1. Experimental verification of multi-feature hierarchical clustering algorithm based on Laplacian matrix
[0085] In order to prove the effectiveness of the clustering algorithm, a two-way lane traffic video image (such as Image 6 The moving target in a) is tracked by a two-dimensional target tracking algorithm with a frame rate of 25 frames / s and an image resolution of 320×240. A total of 296 vehicle trajectories are collected, and 216 effective trajectories are retained after preprocessing such as smoothing ,like Image 6 as shown in b.
[0086] Using the proposed clustering algorithm, the training trajectory is firstly clustered based on the direction of motion, and the clustering results are as follows: Figure 7 As shown in a, the trajectories are roughly divided into two types with opposite directions of motion. O 1 and O 2 ; Then each intermediate cluster is finely clustered based on spatial location, and the final clustering result is as follows Figure 7...
PUM
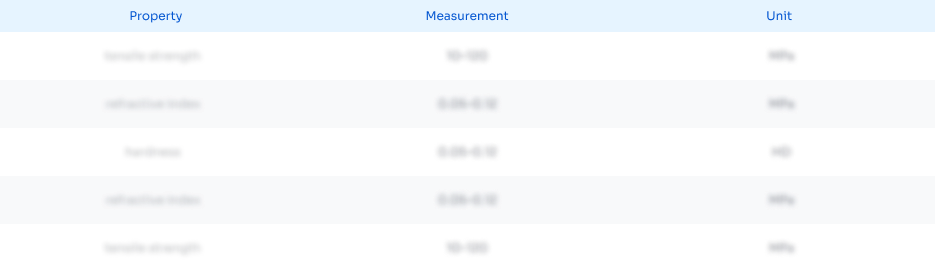
Abstract
Description
Claims
Application Information
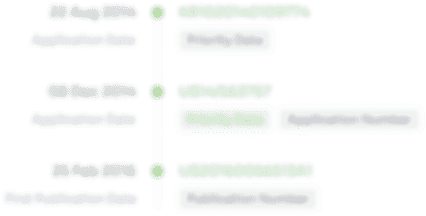
- R&D Engineer
- R&D Manager
- IP Professional
- Industry Leading Data Capabilities
- Powerful AI technology
- Patent DNA Extraction
Browse by: Latest US Patents, China's latest patents, Technical Efficacy Thesaurus, Application Domain, Technology Topic, Popular Technical Reports.
© 2024 PatSnap. All rights reserved.Legal|Privacy policy|Modern Slavery Act Transparency Statement|Sitemap|About US| Contact US: help@patsnap.com