Methods, computer-accessible medium and systems to model disease progression using biomedical data from multiple patients
a disease progression and patient technology, applied in the field of cancer progression models, can solve the problems of not being able to achieve every order, the causal and temporal relationship among the genetic events driving cancer progression remains largely unknown, and the impact of large-scale effects
- Summary
- Abstract
- Description
- Claims
- Application Information
AI Technical Summary
Benefits of technology
Problems solved by technology
Method used
Image
Examples
case iii
[0351]
[0352]The derivation below shows, just like in the DMPN, that θ+> for all true parents sets in the XMPN. The reasoning behind this can be similar to that above, except for the summation can be over the rows in which exactly one parent takes value 1 and the rest take value 0. To denote this, the standard notation Pai(X) can be used to mean the ith parent of X and Pa−i(X) to mean all parents except for the ith parent of X.
[0353]Lemma 3 (Consistency of Polaris):
[0354]Polaris can be a statistically consistent score.
[0355]Exemplary Proof:
[0356]Let M be the number of samples generated by the graph G*=(V, E*). Let G=(V, E) be the graph learned by maximizing the Polaris score, and GBIC be the graph learned by maximizing the BIC score, both for a sufficiently large M. The exemplary Polaris score can consist of three terms: (i) the log-likelihood (LL) term, (ii) the regularization term from BIC and (iii) the monotonicity term. Each of these terms can grow at different rates. The LL term...
case vi
[0368]
[0369]Xi has 2 or more parents. Because G* has no transitive edges, there cannot be any edge between any two parents of Xi. Thus, the parents of Xi can be unwed and form a v-structure with Xi. Because Polaris can be consistent, this v-structure can be learned correctly.
[0370]Exemplary Corollary 1 (Convergence Conditions for Polaris with Filtering):
[0371]For a sufficiently large sample size, M, under the assumptions of no transitive edges and faithful temporal priority relations, filtering with the α-filter and then optimizing Polaris convergences to the exact structure for MPNs. Proof:
[0372]In Lemma 1, it was shown that α-filtering removes no true parent sets. In Theorem 6, it was shown that given a hypothesis space that includes the true parent sets, optimizing Polaris returns the true graph. Because the α-filter does not remove the true parent sets from the hypothesis space, optimizing Polaris will still return the correct structure on the filtered hypothesis space.
[0373]FIG...
PUM
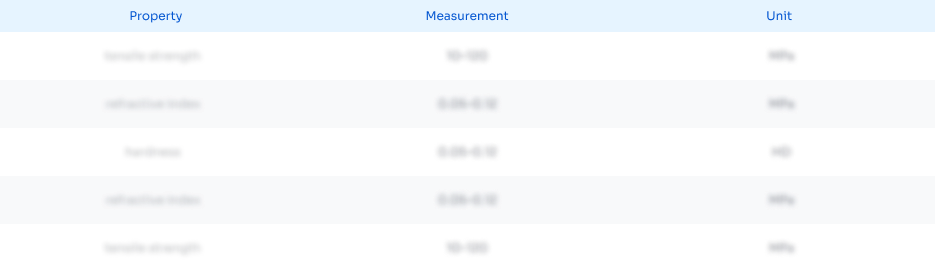
Abstract
Description
Claims
Application Information
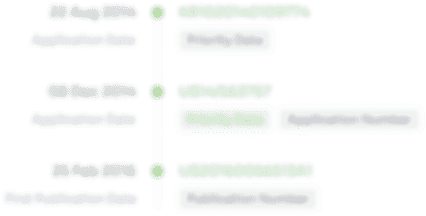
- R&D Engineer
- R&D Manager
- IP Professional
- Industry Leading Data Capabilities
- Powerful AI technology
- Patent DNA Extraction
Browse by: Latest US Patents, China's latest patents, Technical Efficacy Thesaurus, Application Domain, Technology Topic, Popular Technical Reports.
© 2024 PatSnap. All rights reserved.Legal|Privacy policy|Modern Slavery Act Transparency Statement|Sitemap|About US| Contact US: help@patsnap.com