Method, system and apparatus for real-time classification of muscle signals from self -selected intentional movements
a self-selected intentional movement and muscle signal technology, applied in the field of muscle signal real-time classification, can solve the problems of requiring resting contraction, limiting limiting the practical and theoretical limits of increasing the number of channels, so as to improve the ability to connect, improve the operation of such devices, and optimize the performance of the prosthetic device.
- Summary
- Abstract
- Description
- Claims
- Application Information
AI Technical Summary
Benefits of technology
Problems solved by technology
Method used
Image
Examples
example 1
A. Participants
[0140]In a study, 8 able-bodied adults and 1 below-elbow amputee were recruited. Able-bodied subjects consisted of a convenience sample of adults aged 20-30 years with no upper limb deficiencies. An amputee was recruited from a local rehabilitation center. It was particularly desirable to choose an amputee who was not a powered prosthesis user since he or she would most likely be unfamiliar with forearm muscle control. This lack of prior training would present a nontrivial challenge to the automatic separation of EMG signals.
[0141]The raw EMG signals were recorded from two sites on the forearm, using pregelled Ag / AgCl GRASS F-E8SD disposable electrodes. The electrodes were placed in pairs with one reference electrode at each site, as discussed below. As the robustness of the algorithm to any skin conditions was to be investigated, no skin preparation was needed. It has been reported that the bulk of the muscle produces higher amount of EMG signals s...
PUM
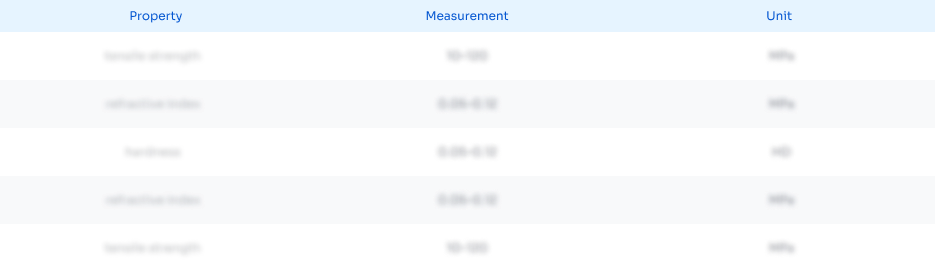
Abstract
Description
Claims
Application Information
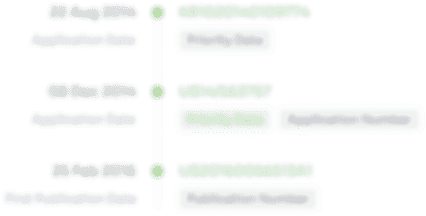
- R&D
- Intellectual Property
- Life Sciences
- Materials
- Tech Scout
- Unparalleled Data Quality
- Higher Quality Content
- 60% Fewer Hallucinations
Browse by: Latest US Patents, China's latest patents, Technical Efficacy Thesaurus, Application Domain, Technology Topic, Popular Technical Reports.
© 2025 PatSnap. All rights reserved.Legal|Privacy policy|Modern Slavery Act Transparency Statement|Sitemap|About US| Contact US: help@patsnap.com