Coder-decoder-based deep learning multi-step irradiance prediction method
A technology of codec and prediction method, applied in the field of deep learning multi-step irradiance prediction, can solve the problem of limited time-dependent feature extraction ability, deep learning model difficult to take into account long-sequence input and long-term dependence, difficult to deal with long input sequence and other problems, to achieve the effect of high-precision irradiance prediction effect
- Summary
- Abstract
- Description
- Claims
- Application Information
AI Technical Summary
Problems solved by technology
Method used
Image
Examples
Embodiment Construction
[0063] In order to illustrate the technical solutions of the present invention more clearly, the present invention is described below with reference to the accompanying drawings. The examples are only used to explain the present invention, not to limit the scope of the present invention.
[0064] refer to figure 1 , an encoder-decoder-based deep learning multi-step irradiance prediction method, comprising the following steps:
[0065] S1, training data acquisition, acquisition of historical irradiance data and corresponding meteorological data in the target area and production of a supervision data set, further, S1 includes the following contents:
[0066] (1) obtain the historical irradiance data of target area and its corresponding meteorological data, in the present embodiment, select temperature T, humidity H, air pressure P, wind speed W etc. as meteorological data;
[0067] (2) If a certain segment of the historical data is missing or illegal, the mean value of the adj...
PUM
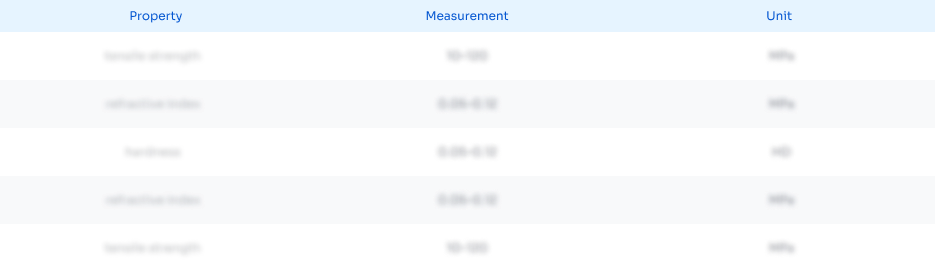
Abstract
Description
Claims
Application Information
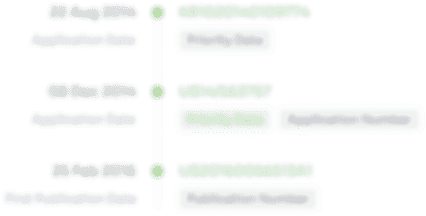
- R&D Engineer
- R&D Manager
- IP Professional
- Industry Leading Data Capabilities
- Powerful AI technology
- Patent DNA Extraction
Browse by: Latest US Patents, China's latest patents, Technical Efficacy Thesaurus, Application Domain, Technology Topic.
© 2024 PatSnap. All rights reserved.Legal|Privacy policy|Modern Slavery Act Transparency Statement|Sitemap