Bayesian tensor completion algorithm based on multiple measurement values
A measurement value, Bayesian technology, applied in the field of tensor completion, can solve the problems of low measurement accuracy and high cost, and achieve the effect of accurate estimation and accurate data completion
- Summary
- Abstract
- Description
- Claims
- Application Information
AI Technical Summary
Problems solved by technology
Method used
Image
Examples
Embodiment Construction
[0051] In order to make the technical means, creative features, goals and effects realized by the present invention easy to understand, the following describes the Bayesian tensor completion algorithm based on multi-measurement values of the present invention in detail with reference to the embodiments and the accompanying drawings.
[0052]
[0053] This embodiment provides a Bayesian tensor completion algorithm based on multiple measurement values, which combines CP decomposition and Gibbs sampling to perform tensor completion on data with multiple measurement values. The data are nuclear physics scattering data, obtained through multiple measurements. The experimental measurement principle is as follows Figure 4 As shown, due to the high cost of measurement and other reasons, the data with multiple measurement values has missing values.
[0054] At the same time, in this embodiment, a Bayesian tensor completion algorithm based on multi-measurement values is impleme...
PUM
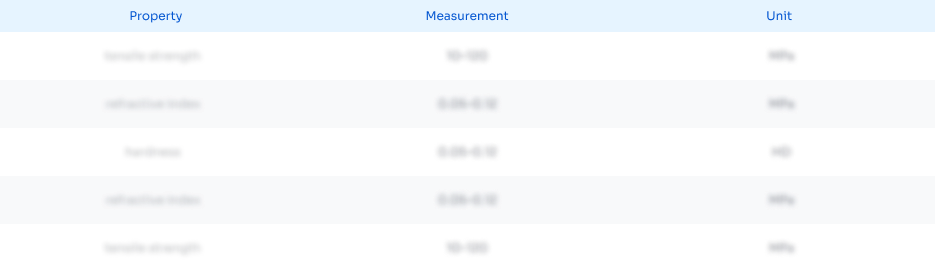
Abstract
Description
Claims
Application Information
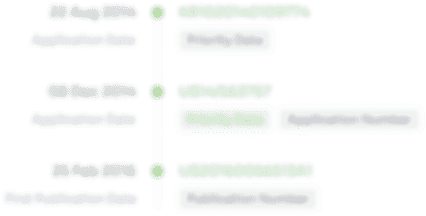
- R&D Engineer
- R&D Manager
- IP Professional
- Industry Leading Data Capabilities
- Powerful AI technology
- Patent DNA Extraction
Browse by: Latest US Patents, China's latest patents, Technical Efficacy Thesaurus, Application Domain, Technology Topic, Popular Technical Reports.
© 2024 PatSnap. All rights reserved.Legal|Privacy policy|Modern Slavery Act Transparency Statement|Sitemap|About US| Contact US: help@patsnap.com