Urban gas daily load prediction method based on GRA-ABC-BPNN
A GRA-ABC-BP, daily load technology, applied in the field of urban gas daily load forecasting, can solve the problems of random initialization weights and thresholds easily falling into local minimum state, unstable prediction results, low learning efficiency, etc., to ensure The effect of gas supply, faster computing speed, and faster convergence speed
- Summary
- Abstract
- Description
- Claims
- Application Information
AI Technical Summary
Problems solved by technology
Method used
Image
Examples
Embodiment Construction
[0036] The following is attached figure 1 The inventive method is described in further detail:
[0037] The flow chart of the city gas daily load forecasting method based on the GRA-ABC-BP neural network model is attached. figure 1 , the forecasting process of the system includes the following steps:
[0038] Step 1: Quantization of non-numerical data. Among the 11 influencing factors, there are 3 non-numerical factors, namely the weather type of the day, the heating situation and the date type. Divide weather types into 7 categories, represented by 1-7; heating conditions are divided into heating and non-heating, represented by 1 and 2 respectively; date types are divided into weekdays, weekends and holidays, represented by 1, 2 and 3 respectively express.
[0039] Calculate the correlation degree between each influencing factor and the daily gas load. The formula is as follows:
[0040]
[0041]
[0042] Among them, ξ i (k) is the comparison sequence x i The gr...
PUM
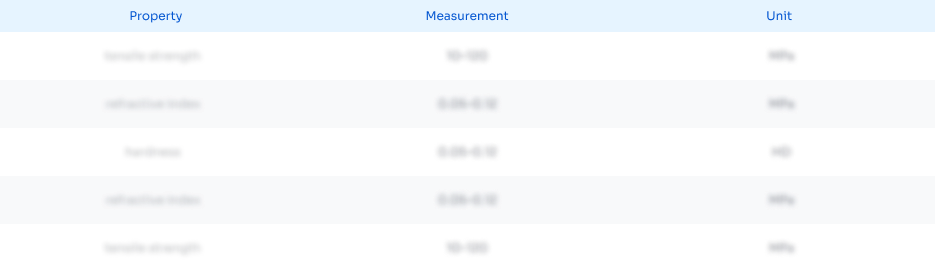
Abstract
Description
Claims
Application Information
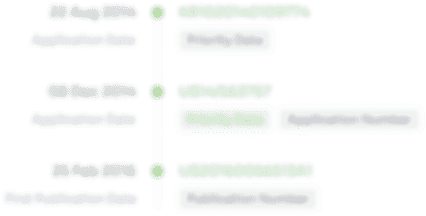
- Generate Ideas
- Intellectual Property
- Life Sciences
- Materials
- Tech Scout
- Unparalleled Data Quality
- Higher Quality Content
- 60% Fewer Hallucinations
Browse by: Latest US Patents, China's latest patents, Technical Efficacy Thesaurus, Application Domain, Technology Topic, Popular Technical Reports.
© 2025 PatSnap. All rights reserved.Legal|Privacy policy|Modern Slavery Act Transparency Statement|Sitemap|About US| Contact US: help@patsnap.com