Power grid real-time adaptive decision-making method based on deep reinforcement learning
A technology of reinforcement learning and decision-making method, applied in the field of deep reinforcement learning, which can solve the problem of new energy consumption without fully considering the robust operation of the new power grid, and cannot solve the problem of explosive action space of the new power grid system optimization strategy exploration vulnerability Problems, no identical or similar problems were found, and good control effects were achieved
- Summary
- Abstract
- Description
- Claims
- Application Information
AI Technical Summary
Problems solved by technology
Method used
Image
Examples
Embodiment Construction
[0102] Embodiments of the present invention are described in further detail below:
[0103] A real-time adaptive decision-making method for power grids based on deep reinforcement learning, such as figure 1 shown, including the following steps:
[0104] Step 1. Model the adaptive scheduling problem of the new power system unit as a Markov decision process (Markov Decision Process, MDP);
[0105] The concrete steps of described step 1 include:
[0106] Use a 4-dimensional tuple to describe (S, A, P, R), where S represents the state set of the power grid system, A represents the action set of the power grid system, and P: S×A×S→[0,1] represents the state Transition probability, R:S×A→R means reward mechanism;
[0107] In this embodiment, step 1 involves the construction of an MDP model. Many control decision-making problems in the power grid can be described as MDP models, which are used to solve discrete time-sequence control problems in stochastic dynamic environments, spec...
PUM
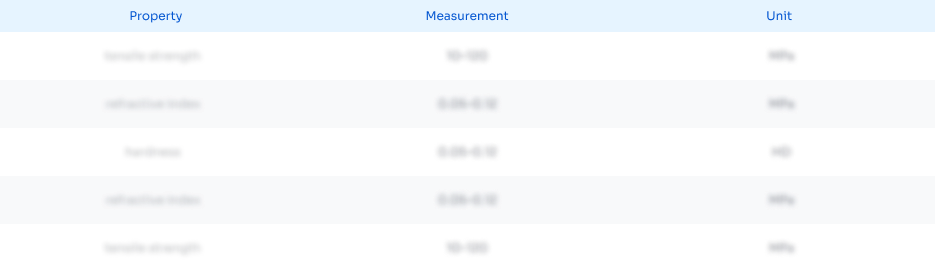
Abstract
Description
Claims
Application Information
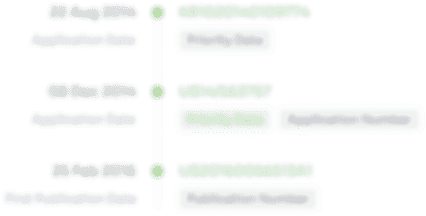
- R&D Engineer
- R&D Manager
- IP Professional
- Industry Leading Data Capabilities
- Powerful AI technology
- Patent DNA Extraction
Browse by: Latest US Patents, China's latest patents, Technical Efficacy Thesaurus, Application Domain, Technology Topic, Popular Technical Reports.
© 2024 PatSnap. All rights reserved.Legal|Privacy policy|Modern Slavery Act Transparency Statement|Sitemap|About US| Contact US: help@patsnap.com