Conditional generative adversarial-based three-dimensional model fuzzy texture feature saliency method
A conditional generation, three-dimensional model technology, applied in biological neural network models, neural learning methods, 3D modeling, etc., can solve the problems of blurred texture boundaries, blurred boundary textures, limited input data clarity, etc., to improve the degree of fidelity , the effect of reducing computation time
- Summary
- Abstract
- Description
- Claims
- Application Information
AI Technical Summary
Problems solved by technology
Method used
Image
Examples
Embodiment Construction
[0028] Such as figure 1 A saliency method for 3D model fuzzy texture features based on conditional generation confrontation is shown, including the following steps:
[0029] Step 1) generate the shape and texture of the three-dimensional geometric model, and obtain the point cloud features of the three-dimensional geometric model;
[0030] First, input the image to HMR (Human Mesh Recovery) and predict the SMPL (Skinned Multi-Person Linear Model) parameters, where HMR uses an iterative 3D regression module to generate shape, pose and translation parameters for SMPL, and the estimated 3D grid m of the input image Expressed as: m=M(β,θ,γ), secondly, use U-Net to generate textures, and use Opendr to render tensors, map the generated textures to 3D grids, use the UV correspondence provided by SMPL and Opendr's The rendering function R(m,t) assigns pixels to the surface of the 3D geometric model, and at the same time uses linear interpolation to fill the gaps, and finally obtains ...
PUM
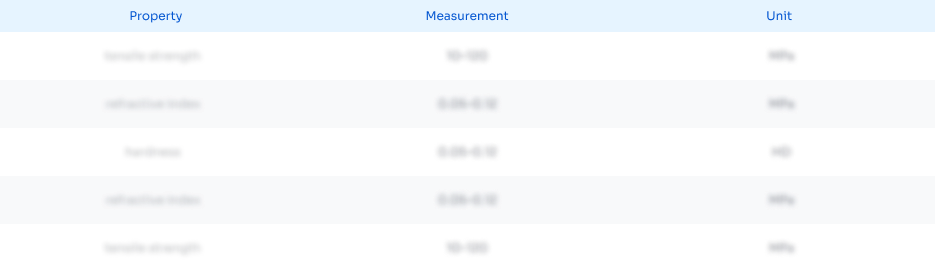
Abstract
Description
Claims
Application Information
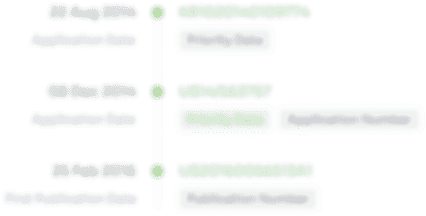
- R&D Engineer
- R&D Manager
- IP Professional
- Industry Leading Data Capabilities
- Powerful AI technology
- Patent DNA Extraction
Browse by: Latest US Patents, China's latest patents, Technical Efficacy Thesaurus, Application Domain, Technology Topic, Popular Technical Reports.
© 2024 PatSnap. All rights reserved.Legal|Privacy policy|Modern Slavery Act Transparency Statement|Sitemap|About US| Contact US: help@patsnap.com