Context sensing recommendation method based on deep neural network
A technology of deep neural network and recommendation method, applied in the field of context-aware recommendation based on deep neural network, can solve problems such as no exact method for problem solving, high-dimensional problem of data features, data coefficient, poor scalability, etc. Solve the cold start problem, no sparse problem, avoid the effect of data sparse
- Summary
- Abstract
- Description
- Claims
- Application Information
AI Technical Summary
Problems solved by technology
Method used
Image
Examples
Embodiment Construction
[0099] An embodiment of the present invention will be further described below in conjunction with the accompanying drawings.
[0100] In the embodiment of the present invention, a context-aware recommendation method based on a deep neural network, on the basis of various collaborative filtering recommendation methods, proposes a new linear graph model, such as figure 1 As shown, the user and the recommended object are described as user feature vectors and recommended object feature vectors, which do not require professional and private information, and have the characteristics of security and simplicity. Each grid is connected to a user and a recommended object, indicating the user's rating on the recommended object, and a high score indicates that the user's feature vector and the recommended object's feature vector have some of the same "interest" "Point" is the recommended object that should be recommended to the user. The user's rating of the recommended object constrains ...
PUM
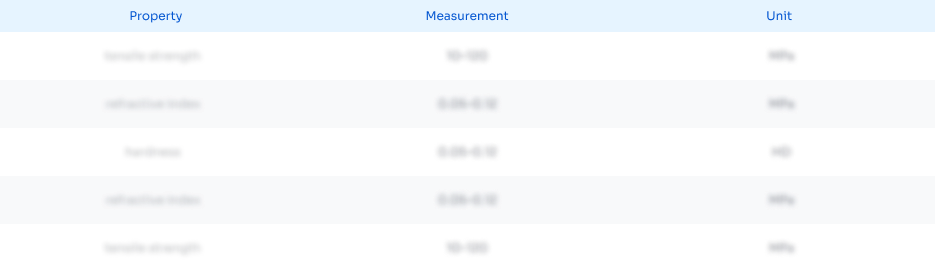
Abstract
Description
Claims
Application Information
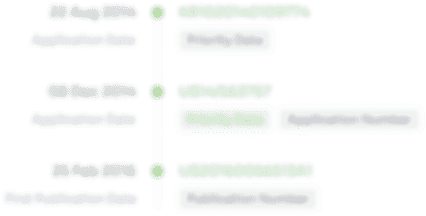
- R&D Engineer
- R&D Manager
- IP Professional
- Industry Leading Data Capabilities
- Powerful AI technology
- Patent DNA Extraction
Browse by: Latest US Patents, China's latest patents, Technical Efficacy Thesaurus, Application Domain, Technology Topic.
© 2024 PatSnap. All rights reserved.Legal|Privacy policy|Modern Slavery Act Transparency Statement|Sitemap