Classification method for airborne system software defects
A technology for software defects and airborne systems, applied in text database clustering/classification, natural language data processing, special data processing applications, etc., can solve the problem of large manpower and resources, affecting airborne software, and airborne software testing efficiency Inefficient and other problems, to achieve the effect of improving efficiency and quality, accurate classification and prediction
- Summary
- Abstract
- Description
- Claims
- Application Information
AI Technical Summary
Problems solved by technology
Method used
Image
Examples
Embodiment Construction
[0071] (1) Implementation preparation
[0072] The object selected in this example is a certain type of airborne display and control software test question list, with a total of 90 items. In advance, according to the functions of various test question sheets, they are manually classified into 9 categories, forming a standard classification set, that is, these 90 test question sheets belong to 9 functions on average.
[0073] The data classification methods used for comparison include: the traditional LDA topic model (denoted as LDA-CF), the improved LDA topic model (denoted as RadarDCP-CF) proposed by the present invention and integrated into the airborne software requirements feature, and the mainstream classification algorithm support vector Machine SVM (denoted as SVM-CF).
[0074] The present invention uses the python language to realize the LDA topic model under the Windows7 operating system. The main parameters of the LDA topic model are set as follows: Gibbs sampling ...
PUM
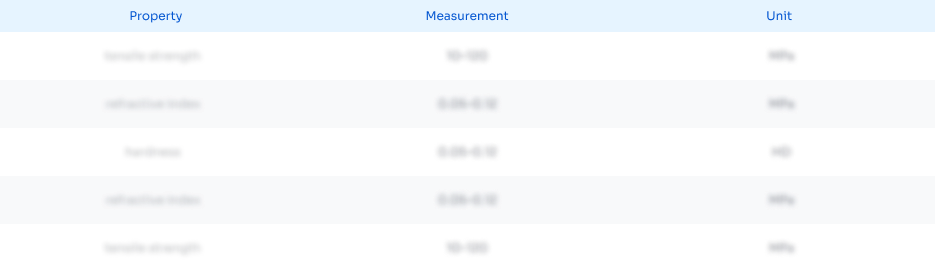
Abstract
Description
Claims
Application Information
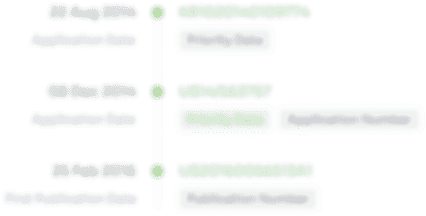
- R&D Engineer
- R&D Manager
- IP Professional
- Industry Leading Data Capabilities
- Powerful AI technology
- Patent DNA Extraction
Browse by: Latest US Patents, China's latest patents, Technical Efficacy Thesaurus, Application Domain, Technology Topic, Popular Technical Reports.
© 2024 PatSnap. All rights reserved.Legal|Privacy policy|Modern Slavery Act Transparency Statement|Sitemap|About US| Contact US: help@patsnap.com