Machine learning model adjustment method and device based on ensemble learning
A machine learning model and ensemble learning technology, applied in the field of machine learning, can solve the problems of small data volume, noise, and output easily affected by noise points, etc., to improve performance and accuracy.
- Summary
- Abstract
- Description
- Claims
- Application Information
AI Technical Summary
Problems solved by technology
Method used
Image
Examples
Embodiment Construction
[0029] In order to make the purpose, technical solution and advantages of the present invention clearer, the embodiments of the present invention will be further described below in conjunction with the accompanying drawings.
[0030] Please refer to figure 1 , figure 1 It is a schematic flow chart of the method of the present invention; a kind of machine learning model adjustment method and equipment based on integrated learning provided by the present invention comprises the following steps:
[0031] S101: Construct an initial classification model; the initial classification model is a machine learning model;
[0032] As an embodiment, the machine learning model may be: machine learning models such as kernel SVM, LR and RC;
[0033] S102: Using the BF method to perform k rounds of iterative adjustment on the initial classification model to reduce the deviation of the initial classification model;
[0034] As an example, please refer to figure 2 , figure 2 is a schematic ...
PUM
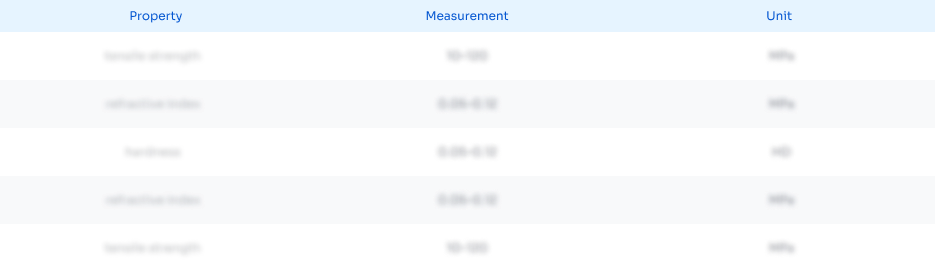
Abstract
Description
Claims
Application Information
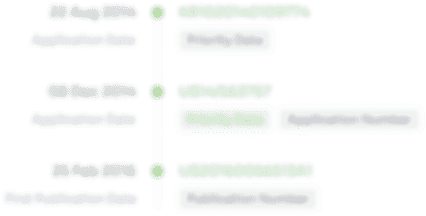
- R&D Engineer
- R&D Manager
- IP Professional
- Industry Leading Data Capabilities
- Powerful AI technology
- Patent DNA Extraction
Browse by: Latest US Patents, China's latest patents, Technical Efficacy Thesaurus, Application Domain, Technology Topic, Popular Technical Reports.
© 2024 PatSnap. All rights reserved.Legal|Privacy policy|Modern Slavery Act Transparency Statement|Sitemap|About US| Contact US: help@patsnap.com