Drill bit wear quantitative evaluation method suitable for machine learning
A technology of machine learning and quantitative evaluation, applied in the field of drilling engineering, can solve problems such as difficulties, no reference, and low use value, and achieve the effects of avoiding severe wear, prolonging service life, and optimizing drilling parameters
- Summary
- Abstract
- Description
- Claims
- Application Information
AI Technical Summary
Problems solved by technology
Method used
Image
Examples
Embodiment 1
[0030] The invention provides a method for quantitative evaluation of drill bit wear suitable for machine learning, comprising the following steps:
[0031] Step 1) Regional data collection, based on the data of 34 wells drilled in a certain block of the Bohai Sea in recent years, combined with regional experience and actual needs, the selection of influencing factors is: bit type, suspension weight, weight on bit, bit speed, etc. 13 Three factors are used as input features, which comprehensively reflect practical problems such as drilling engineering parameters, mud performance, and early wear factors of drill bits, and the evaluation of drill bit wear is taken as the analysis target.
[0032]
[0033]
[0034] Step 2) The engineering data set of 34 wells in step 1) is used as a label to make a data set according to the drill bit wear evaluation, which can be described as {(x(i), y(i))} (i=1, 2 ,···,m), where x(i)∈R n is the feature vector of the i-th sample, and y(i) ...
PUM
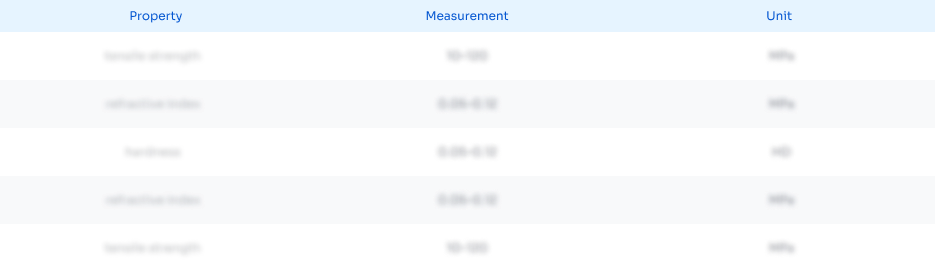
Abstract
Description
Claims
Application Information
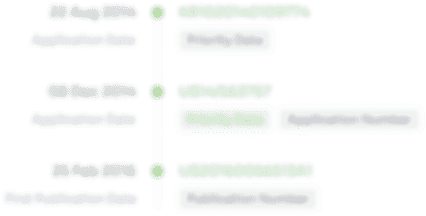
- R&D Engineer
- R&D Manager
- IP Professional
- Industry Leading Data Capabilities
- Powerful AI technology
- Patent DNA Extraction
Browse by: Latest US Patents, China's latest patents, Technical Efficacy Thesaurus, Application Domain, Technology Topic, Popular Technical Reports.
© 2024 PatSnap. All rights reserved.Legal|Privacy policy|Modern Slavery Act Transparency Statement|Sitemap|About US| Contact US: help@patsnap.com