A Traffic Prediction Method for Communication Base Station
A communication base station and flow prediction technology, which is applied in wireless communication, artificial life, electrical components, etc., can solve the problems of large communication base station flow prediction error and difficulty in effectively capturing nonlinear factors, so as to reduce the risk of convergence and balance the overall situation Search ability and local development, the effect of improving local development ability
- Summary
- Abstract
- Description
- Claims
- Application Information
AI Technical Summary
Problems solved by technology
Method used
Image
Examples
specific Embodiment approach 1
[0067] This embodiment is a communication base station flow prediction method based on the improved gray wolf algorithm to optimize the support vector machine regression machine.
[0068] The present invention firstly collects the flow data of the communication base station and divides and processes them; secondly, sets the relevant parameters of SVR and sets the parameters of the improved gray wolf optimization algorithm; Finally, the optimized SVR is used to predict the test set data, and the output results are denormalized to obtain the prediction results of communication base station traffic. The present invention improves the standard gray wolf algorithm for SVR parameter optimization. Compared with the standard gray wolf algorithm optimized support vector machine regression machine prediction model, simulated annealing algorithm optimized BP neural network prediction model and other models in base station traffic prediction have higher prediction accuracy.
[0069] Aimi...
Embodiment
[0150] Carry out simulation based on the present invention, compare existing model simultaneously, apply the experimental result of the present invention and all the other three kinds of model results as follows:
[0151] In order to verify the high prediction accuracy of the LIGWO_SVR prediction model proposed by the present invention, the prediction results of the model are compared with the prediction results of the GWO_SVR model, the prediction results of the SA_BP model, the results of the PSO_BP model and the actual communication base station traffic data. The rest of the model parameters are set as follows: the Markov chain length of the SA_BP model is L=10, the initial temperature T ini =8, final temperature T fin =3, decay parameter Dec=0.85, Metropolis step factor M=0.2. PSO_BP model population size N=50, the maximum number of iterations t max =100, individual learning factor c1=1.49, social learning factor c2=1.49, inertia factor ω=0.2. The number of hidden layer...
PUM
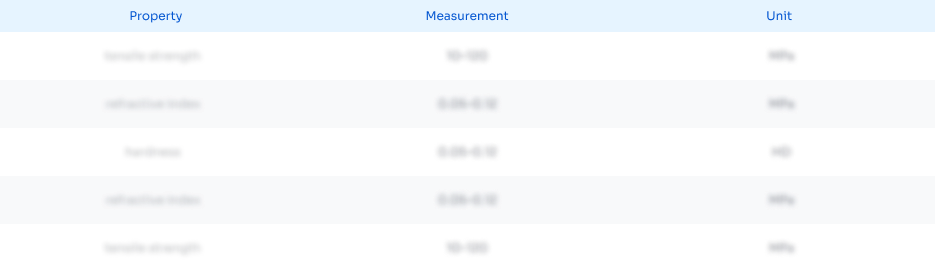
Abstract
Description
Claims
Application Information
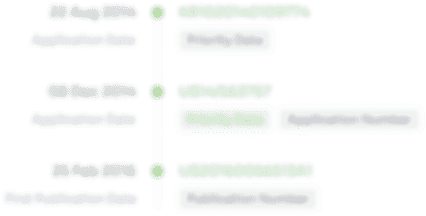
- R&D Engineer
- R&D Manager
- IP Professional
- Industry Leading Data Capabilities
- Powerful AI technology
- Patent DNA Extraction
Browse by: Latest US Patents, China's latest patents, Technical Efficacy Thesaurus, Application Domain, Technology Topic, Popular Technical Reports.
© 2024 PatSnap. All rights reserved.Legal|Privacy policy|Modern Slavery Act Transparency Statement|Sitemap|About US| Contact US: help@patsnap.com