Apple grading method and system based on residual network
A classification method, Apple's technology, applied in image analysis, image data processing, instruments, etc., to achieve the effect of alleviating the problem of sudden failure, easy key information, and easy extraction
- Summary
- Abstract
- Description
- Claims
- Application Information
AI Technical Summary
Problems solved by technology
Method used
Image
Examples
Embodiment 1
[0055] Such as figure 1 As shown, the present disclosure provides a residual network-based apple grading method including:
[0056] Get the appearance image of the apple;
[0057] According to the obtained appearance image and the preset apple grading network model, the apple grading result is obtained;
[0058] Among them, the Apple hierarchical network model is obtained by training the improved ResNet-50 network; specifically, the improvement of the ResNet-50 network is realized by adding a convolutional attention module and a leaky linear rectification function.
[0059] In this embodiment, the training process of Apple's hierarchical network model is:
[0060] Obtain the appearance image of the apple to obtain the training set; each apple is measured multiple times, and the appearance image of the apple is obtained from multiple sides and different positions on the top surface;
[0061] Data augmentation of appearance images in the training set, including vertical mirro...
Embodiment 2
[0103] In order to verify the effect of this scheme, in this embodiment, the apple grading method based on the residual network proposed in the embodiment 1 is experimentally verified, specifically:
[0104] The red Fuji apple data used in the present embodiment comprises 6759 effective pictures; After the selection of grading standards, a total of 781 special-class, 1,241 first-class, and 1,032 second-class were sorted out.
[0105] Table 1 Grading standard of Red Fuji apple
[0106]
[0107] The above data is randomly divided into training set and verification set according to the ratio of 8:2. The apple grading dataset is shown in Table 2.
[0108] Table 2 Apple Grading Dataset
[0109]
[0110] The experiment was carried out under the Ubuntu 18.04 system, and two 2080Ti graphics card GPUs were used to accelerate the training of the model.
[0111] In order to verify the effectiveness and applicability of the improved ResNet-50 network in the Apple grading system,...
Embodiment 3
[0119] The present embodiment provides a kind of apple grading system based on residual network, including image acquisition module and grading module;
[0120] The image acquisition module is configured to: acquire an appearance image of an apple;
[0121] The grading module is configured to: obtain an apple grading result according to the acquired appearance image and the preset apple grading network model;
[0122] Among them, the Apple hierarchical network model is obtained by training the improved ResNet-50 network; specifically, the improvement of the ResNet-50 network is realized by adding a convolutional attention module and a leaky rectification function.
PUM
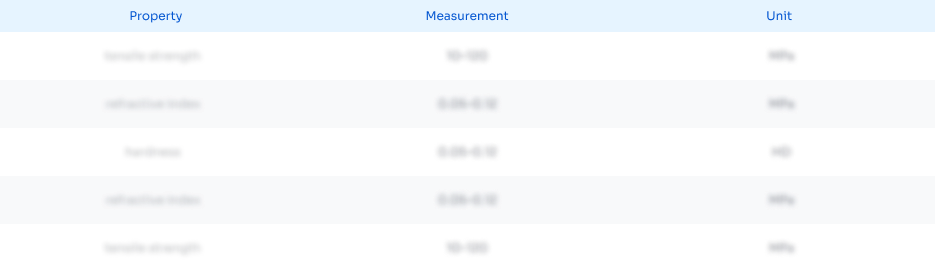
Abstract
Description
Claims
Application Information
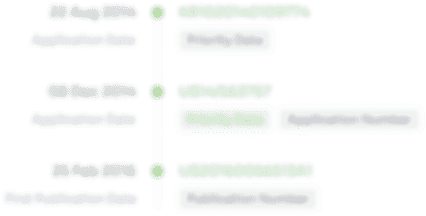
- R&D Engineer
- R&D Manager
- IP Professional
- Industry Leading Data Capabilities
- Powerful AI technology
- Patent DNA Extraction
Browse by: Latest US Patents, China's latest patents, Technical Efficacy Thesaurus, Application Domain, Technology Topic, Popular Technical Reports.
© 2024 PatSnap. All rights reserved.Legal|Privacy policy|Modern Slavery Act Transparency Statement|Sitemap|About US| Contact US: help@patsnap.com