Equipment fault prediction method based on DCFM model
A technology for equipment failure and prediction methods, applied in computing models, neural learning methods, biological neural network models, etc., can solve the problems of ignoring important information, increasing the computational complexity of the model, and affecting the prediction effect.
- Summary
- Abstract
- Description
- Claims
- Application Information
AI Technical Summary
Problems solved by technology
Method used
Image
Examples
Embodiment Construction
[0034] A specific embodiment is given below, and a better understanding of the technical solution and the achieved effects of the present invention can be obtained in combination with the embodiment.
[0035] The data set used in the embodiment comes from the smart device project implemented by the experimental team in 2018. Table 1 shows the 16-dimensional features in the original data set of the device. In order to reduce the impact of feature redundancy on the efficiency of model training, the XGBoost algorithm is used to screen the original device features , retains important 10-dimensional features, as shown in Table 2, mainly including equipment status, power, gearbox inlet oil temperature, gearbox oil temperature, engine room temperature, wind speed, impeller speed, hydraulic oil temperature at yaw position, etc.
[0036] Table 1. Raw characteristics of equipment failure
[0037]
[0038]
[0039] Table 2. Features and descriptions after equipment failure screenin...
PUM
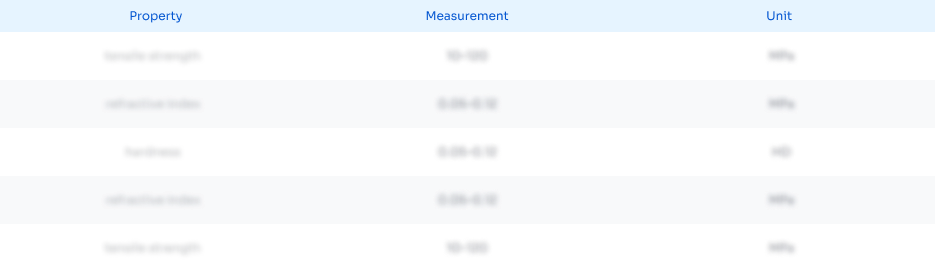
Abstract
Description
Claims
Application Information
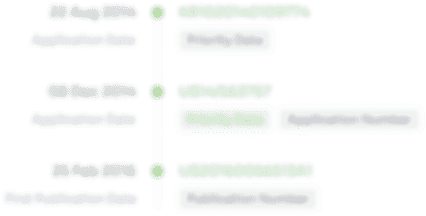
- R&D Engineer
- R&D Manager
- IP Professional
- Industry Leading Data Capabilities
- Powerful AI technology
- Patent DNA Extraction
Browse by: Latest US Patents, China's latest patents, Technical Efficacy Thesaurus, Application Domain, Technology Topic, Popular Technical Reports.
© 2024 PatSnap. All rights reserved.Legal|Privacy policy|Modern Slavery Act Transparency Statement|Sitemap|About US| Contact US: help@patsnap.com