Multi-target regression method based on multi-class multi-label evolution super network
A regression method and super-network technology, applied in general water supply conservation, design optimization/simulation, complex mathematical operations, etc., can solve problems such as ignoring the specificity of the output target, nonlinearity, etc.
- Summary
- Abstract
- Description
- Claims
- Application Information
AI Technical Summary
Problems solved by technology
Method used
Image
Examples
Embodiment 1
[0053] In order to further illustrate the solution of the present invention, the Andro data set in the multi-objective regression problem is used to elaborate the solution of the present invention. The Andro data set contains 49 data samples, each sample is described by 30 features, corresponding to 6 output targets. figure 2 It is a framework diagram of a multi-objective regression method based on multi-class multi-label evolution supernetwork:
[0054] Step 1 converts the multi-objective regression problem into a multi-class multi-label classification problem by clustering:
[0055] First, for each output target in the multi-objective regression data, the one-dimensional Kmeans clustering algorithm is used to cluster into multiple clusters, and the optimal cluster number is adaptively determined by the steepest descent method of cluster deviation. After the clustering is completed, each output target is clustered into multiple clusters, each cluster corresponds to a categor...
PUM
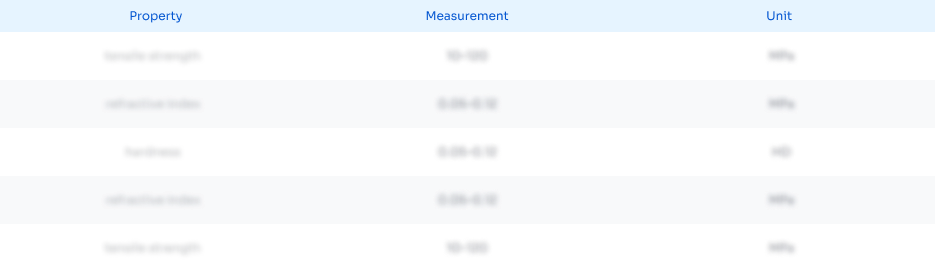
Abstract
Description
Claims
Application Information
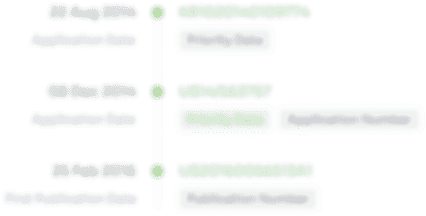
- R&D Engineer
- R&D Manager
- IP Professional
- Industry Leading Data Capabilities
- Powerful AI technology
- Patent DNA Extraction
Browse by: Latest US Patents, China's latest patents, Technical Efficacy Thesaurus, Application Domain, Technology Topic, Popular Technical Reports.
© 2024 PatSnap. All rights reserved.Legal|Privacy policy|Modern Slavery Act Transparency Statement|Sitemap|About US| Contact US: help@patsnap.com