Training method and detection method for generative adversarial multi-relation graph network
A training method and relational graph technology, applied in biological neural network models, neural learning methods, character and pattern recognition, etc., can solve problems such as poor generalization, deepening of mixing, and difficult detection and recognition of machine accounts, so as to improve the detection ability Effect
- Summary
- Abstract
- Description
- Claims
- Application Information
AI Technical Summary
Problems solved by technology
Method used
Image
Examples
Embodiment Construction
[0028] In order to make the object, technical solution and advantages of the present invention clearer, the present invention will be further described in detail below in conjunction with specific embodiments and with reference to the accompanying drawings.
[0029] The embodiment of the present invention discloses a training method for generating an adversarial multi-relational graph network model for detecting machine accounts, wherein the generated adversarial multi-relational graph network model includes a generator G, a connection relationship discriminator D, and a classifier. The method includes: modeling accounts on different platforms as nodes v; modeling interactive operations between accounts as relationships r, wherein the number of relationships r is determined by the number of types of interactive operations between accounts; Model a graph containing nodes and relationships Among them, the figure The number of is determined by the number of the relationship r;...
PUM
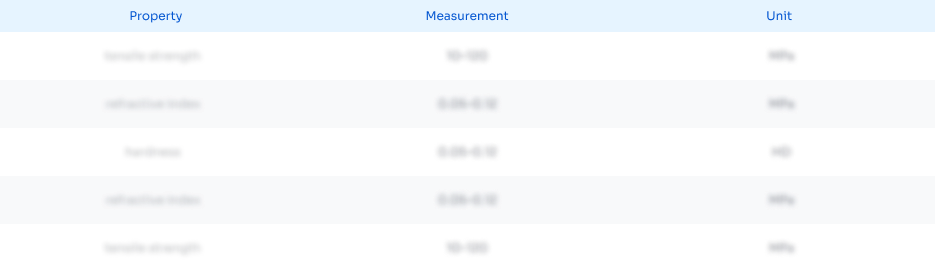
Abstract
Description
Claims
Application Information
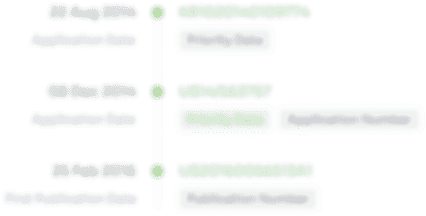
- R&D Engineer
- R&D Manager
- IP Professional
- Industry Leading Data Capabilities
- Powerful AI technology
- Patent DNA Extraction
Browse by: Latest US Patents, China's latest patents, Technical Efficacy Thesaurus, Application Domain, Technology Topic, Popular Technical Reports.
© 2024 PatSnap. All rights reserved.Legal|Privacy policy|Modern Slavery Act Transparency Statement|Sitemap|About US| Contact US: help@patsnap.com