Depression classification method based on graph embedding and multi-modal brain network
A classification method and brain network technology, applied in the field of depression classification based on graph embedding and multimodal brain network, can solve the problem of insufficient feature mining ability of brain network analysis methods, and achieve the effect of improving accuracy
- Summary
- Abstract
- Description
- Claims
- Application Information
AI Technical Summary
Problems solved by technology
Method used
Image
Examples
Embodiment Construction
[0021] The present invention will be further described below in conjunction with the accompanying drawings.
[0022] refer to figure 1 , a depression classification method based on graph embedding and multimodal brain network, including the following steps:
[0023] Step 1: fMRI and DTI data preprocessing: fMRI data preprocessing steps include: removal of the first 10 time points, time correction, head motion correction, image registration, spatial smoothing, filtering and regression For non-neuronal confounding factors, the preprocessing steps of diffusion tensor imaging data include: estimation and correction of distortion caused by magnetic susceptibility, decerebration and eddy current correction;
[0024] Step 2: Build a whole brain functional network: divide the whole brain into 116 brain regions based on the AAL template, and each brain region is a node of the brain network. For the data preprocessed by fMRI, calculate the Time series mean value, and then calculate th...
PUM
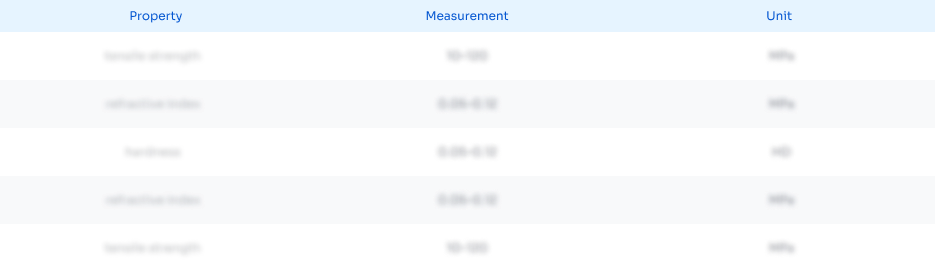
Abstract
Description
Claims
Application Information
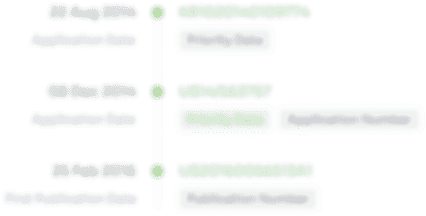
- R&D Engineer
- R&D Manager
- IP Professional
- Industry Leading Data Capabilities
- Powerful AI technology
- Patent DNA Extraction
Browse by: Latest US Patents, China's latest patents, Technical Efficacy Thesaurus, Application Domain, Technology Topic, Popular Technical Reports.
© 2024 PatSnap. All rights reserved.Legal|Privacy policy|Modern Slavery Act Transparency Statement|Sitemap|About US| Contact US: help@patsnap.com