Artificial intelligence data annotation task allocation method and device
A technology of artificial intelligence and task assignment, applied in the direction of instruments, character and pattern recognition, computer components, etc., can solve the problem that it is difficult to find the global task-annotator matching mode, and achieve the effect of improving labeling efficiency and optimizing the labeling process
- Summary
- Abstract
- Description
- Claims
- Application Information
AI Technical Summary
Problems solved by technology
Method used
Image
Examples
Embodiment 1
[0054] refer to figure 1 , which shows a schematic flowchart of a method for assigning an artificial intelligence data labeling task provided by an embodiment of the present application, which is applied to an artificial intelligence data labeling task allocation system.
[0055] Allocation methods for artificial intelligence data labeling tasks include:
[0056] S101: Mark each callable manual annotator as a labeling terminal, and use the personalized information of the manual labeler as a feature vector of the labeling terminal.
[0057] Wherein, the number of the labeling terminals is N, that is, the number of human labelers is N.
[0058] Optionally, the personalized information of the human annotator may be gender, age, place of origin, education, industry, foreign language proficiency, etc.
[0059] It should be noted that different human labelers can achieve different labor productivity for the same labeling task due to differences in factors such as gender, education b...
Embodiment 2
[0105] refer to figure 2 , shows a schematic structural diagram of an artificial intelligence data labeling task allocation device provided in an embodiment of the present application, and the artificial intelligence data labeling task allocation device 20 is applied to a data recommendation system. The artificial intelligence data labeling task distribution device 20 includes:
[0106] The labeling module 201 is used to mark each callable manual labeler as a labeling terminal, and use the personalized information of the manual labeler as the feature vector of the labeling terminal, wherein the number of labeling terminals for N;
[0107] An acquisition module 202, configured to acquire data to be labeled, wherein the data to be labeled includes trial label data and mass production data;
[0108] A sending module 203, configured to equally divide the test label data into N test label sub-data, and send one of the test label sub-data to each of the labeling terminals;
[01...
PUM
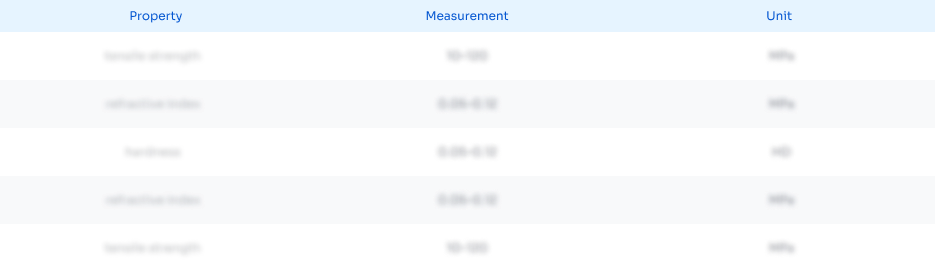
Abstract
Description
Claims
Application Information
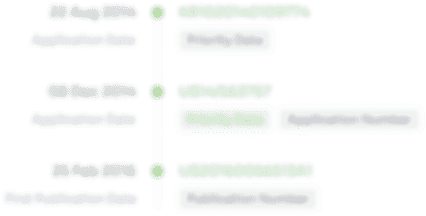
- R&D Engineer
- R&D Manager
- IP Professional
- Industry Leading Data Capabilities
- Powerful AI technology
- Patent DNA Extraction
Browse by: Latest US Patents, China's latest patents, Technical Efficacy Thesaurus, Application Domain, Technology Topic, Popular Technical Reports.
© 2024 PatSnap. All rights reserved.Legal|Privacy policy|Modern Slavery Act Transparency Statement|Sitemap|About US| Contact US: help@patsnap.com