Hyperspectral remote sensing image classification method and system based on multi-saliency feature fusion
A hyperspectral remote sensing and feature fusion technology, which is applied in the field of hyperspectral remote sensing image classification methods and systems, can solve the problems of long training time and difficulty in parameter adjustment, so as to make up for the difficulty of model parameter adjustment, increase the leading role of classification, and improve the utilization of features rate effect
- Summary
- Abstract
- Description
- Claims
- Application Information
AI Technical Summary
Problems solved by technology
Method used
Image
Examples
Embodiment 1
[0053] combined with figure 1 , this embodiment proposes a hyperspectral remote sensing image classification method based on the fusion of multiple salient features, and its implementation includes:
[0054] Step S1, using principal component analysis (PCA) to reduce the dimensionality of the original hyperspectral remote sensing image.
[0055] Step S2, for the hyperspectral remote sensing image after dimensionality reduction, first use the extended morphology method EMP to obtain multiple morphological feature maps, and then use the local binary model LBP and gradient histogram HOG to extract the texture features and gradients of the morphological feature maps feature, and then use the saliency BMS based on Boolean mapping to obtain the saliency feature Ⅰ based on the texture feature and the saliency feature Ⅱ based on the gradient feature. The saliency feature Ⅰ and saliency feature Ⅱ are fused, and the corresponding pixel values are bit by bit Take the average value to ...
Embodiment 2
[0073] This embodiment proposes a hyperspectral remote sensing image classification system based on the fusion of multiple salient features, which includes a dimensionality reduction module 1, an EMP module 2, an LBP module 3, a HOG module 4, a BMS module 5, a fusion module 6, and a fusion module 2.7. Fusion module 3.8. Random forest module 9. combined with figure 2 , in order to better describe the data transfer process between each module, attached figure 2 Two LBP modules 3 and two HOG modules 4 are drawn in the figure. In fact, there is only one LBP module 3 and one HOG module 4 in this system. Attached figure 2 The solid arrows and hollow arrows represent the different processing processes of the original hyperspectral remote sensing image, and the double hollow arrows represent the fusion feature C finally output by the fusion module 38.
[0074] Dimensionality reduction module 1 uses principal component analysis (PCA) to reduce the dimensionality of the original hy...
PUM
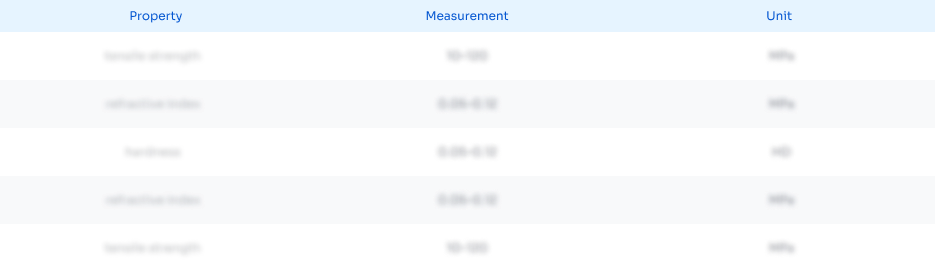
Abstract
Description
Claims
Application Information
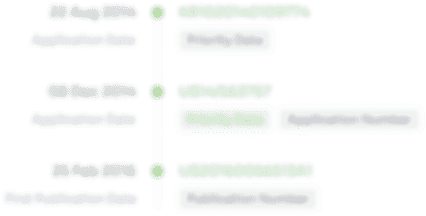
- R&D Engineer
- R&D Manager
- IP Professional
- Industry Leading Data Capabilities
- Powerful AI technology
- Patent DNA Extraction
Browse by: Latest US Patents, China's latest patents, Technical Efficacy Thesaurus, Application Domain, Technology Topic, Popular Technical Reports.
© 2024 PatSnap. All rights reserved.Legal|Privacy policy|Modern Slavery Act Transparency Statement|Sitemap|About US| Contact US: help@patsnap.com