Urban road network short-term traffic flow prediction method based on space-time residual hybrid model
A technology of short-term traffic flow and mixed models, which is applied in forecasting, neural learning methods, biological neural network models, etc., can solve problems such as low practicability, low time series, easy to capture correlation, etc., and achieve high accuracy and practicality performance, reduce training error, and improve prediction accuracy
- Summary
- Abstract
- Description
- Claims
- Application Information
AI Technical Summary
Problems solved by technology
Method used
Image
Examples
Embodiment Construction
[0044] The technical solutions of the present invention will be further described in detail below with reference to the accompanying drawings.
[0045] like Figure 1-2 As shown in the figure, a method for short-term traffic flow prediction of urban road network based on a mixed model of spatio-temporal residual error includes the following steps:
[0046] Step 1) Collect the current latitude and longitude data of the vehicle by taxis, buses and other vehicles equipped with GPS positioning devices and store them in the backend server Hadoop big data cluster database, and utilize the cluster parallel computing component Spark to perform data preprocessing on the original data;
[0047] In the step 1, the longitude and latitude data of taxis, buses and other vehicles equipped with GPS positioning devices are collected, and the original vehicle data set P sorted by time is obtained, P={(P id1 ,P lon1 ,P lat1 ,P time1 ) 1 , (P id2 ,P lon2 ,P lat2 ,P time2 ) 2,…, (P idi ...
PUM
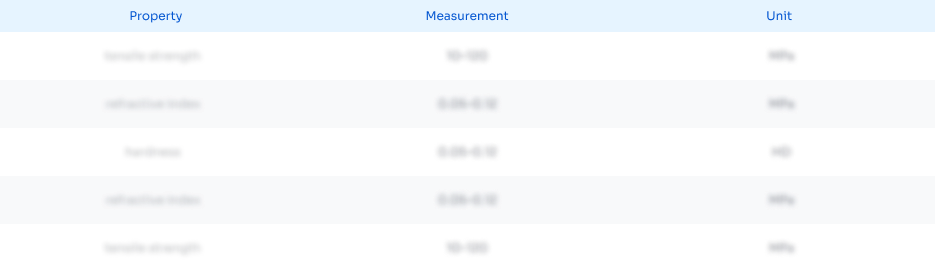
Abstract
Description
Claims
Application Information
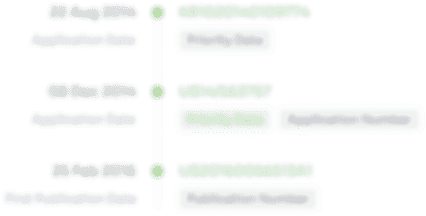
- Generate Ideas
- Intellectual Property
- Life Sciences
- Materials
- Tech Scout
- Unparalleled Data Quality
- Higher Quality Content
- 60% Fewer Hallucinations
Browse by: Latest US Patents, China's latest patents, Technical Efficacy Thesaurus, Application Domain, Technology Topic, Popular Technical Reports.
© 2025 PatSnap. All rights reserved.Legal|Privacy policy|Modern Slavery Act Transparency Statement|Sitemap|About US| Contact US: help@patsnap.com