Modeling method for predicting multi-factor induced landslide based on deep learning
A technology of deep learning and modeling methods, applied in neural learning methods, character and pattern recognition, biological neural network models, etc., can solve problems such as difficult modeling and prediction, single early warning indicators of models, and emphasis on qualitative analysis
- Summary
- Abstract
- Description
- Claims
- Application Information
AI Technical Summary
Problems solved by technology
Method used
Image
Examples
Embodiment 1
[0033] 1. Overview of the research area and data sources
[0034] 1.1 Overview of the research area
[0035] The total area of Hunan Province is about 21.2 square kilometers. The province's landforms are dominated by hills and mountains, accounting for about 80% of the total area. Geological disasters occur frequently. According to statistics, the province's high and medium geological disaster-prone areas reach 164,500 square kilometers, accounting for about 78% of the province's area. As of the end of 2019, a total of 18,496 hidden dangers of geological disasters have been identified in the province, including mudslides, collapses, landslides and ground subsidence, etc., affecting 709,500 people and involving 28.3 billion yuan in property. Among them, the most important types of geological disasters are Rainfall landslide disaster.
[0036] 1.2 Selection and source of data
[0037] From the perspective of disaster science, the causes of landslides include hazard-forming ...
PUM
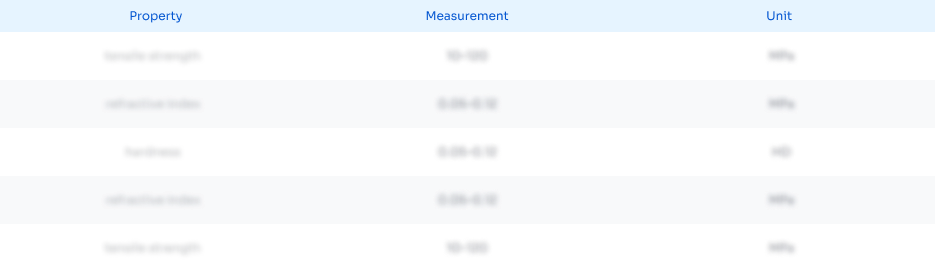
Abstract
Description
Claims
Application Information
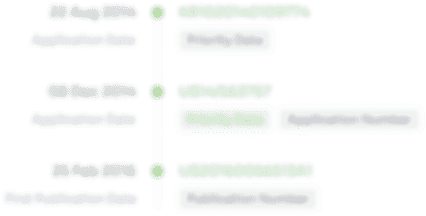
- R&D Engineer
- R&D Manager
- IP Professional
- Industry Leading Data Capabilities
- Powerful AI technology
- Patent DNA Extraction
Browse by: Latest US Patents, China's latest patents, Technical Efficacy Thesaurus, Application Domain, Technology Topic.
© 2024 PatSnap. All rights reserved.Legal|Privacy policy|Modern Slavery Act Transparency Statement|Sitemap