Symbol transfer entropy and brain network feature calculation method based on time-frequency energy
A feature calculation and entropy transfer technology, applied in sensors, diagnostic recording/measurement, medical science, etc., can solve the problems of being easily affected by noise, difficult to obtain the frequency domain features of EEG signals, and slow in calculation speed, so as to improve the accuracy of classification. rate effect
- Summary
- Abstract
- Description
- Claims
- Application Information
AI Technical Summary
Problems solved by technology
Method used
Image
Examples
Embodiment Construction
[0057] The software environment of the concrete experiment of the present invention is: Windows 10 (64 bits), Matlab R2017a.
[0058] The MI-EEG data of the embodiment of the present invention comes from the BCI 2000 public data set, and 64 electrodes under the standard 10-20 system distribution are used to collect EEG data. The electrode distribution positions are as follows figure 2 shown. The sampling frequency of the EEG signal is 160Hz, after 1-50Hz filtering and 50Hz notch filtering. The data set contains a total of 109 subjects. The imaginary task is left-handed or right-handed movement, and each subject performs about 45 experiments. Each experiment lasted about 8 seconds, of which 0 to 4 seconds was the motor imagery period.
[0059] Based on the above-mentioned MI-EEG data set, the specific implementation steps of the present invention are as follows:
[0060] Step 1: Signal preprocessing.
[0061] CAR filtering was performed on the original MI-EEG signal to rem...
PUM
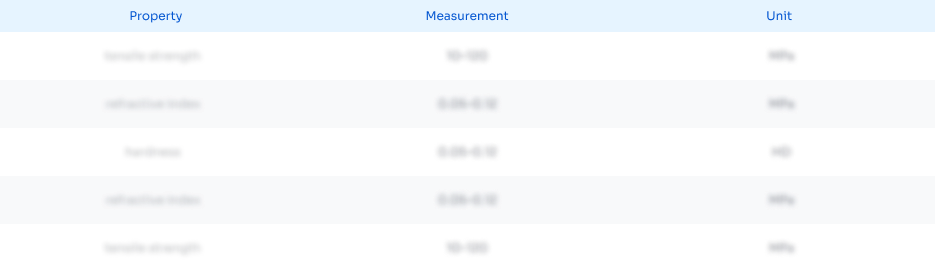
Abstract
Description
Claims
Application Information
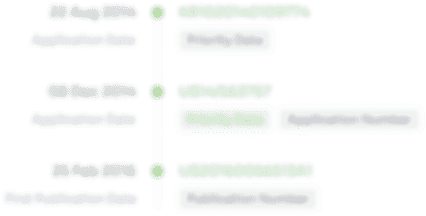
- Generate Ideas
- Intellectual Property
- Life Sciences
- Materials
- Tech Scout
- Unparalleled Data Quality
- Higher Quality Content
- 60% Fewer Hallucinations
Browse by: Latest US Patents, China's latest patents, Technical Efficacy Thesaurus, Application Domain, Technology Topic, Popular Technical Reports.
© 2025 PatSnap. All rights reserved.Legal|Privacy policy|Modern Slavery Act Transparency Statement|Sitemap|About US| Contact US: help@patsnap.com