Semi-supervised industrial product flaw detection method and system based on positive sample learning
A technology for detecting industrial products and defects, applied in the field of image processing
- Summary
- Abstract
- Description
- Claims
- Application Information
AI Technical Summary
Problems solved by technology
Method used
Image
Examples
Embodiment Construction
[0017] This embodiment relates to a semi-supervised defect detection system based on positive sample learning, including: a defect generation module, an image repair network module, a defect segmentation module, an image repair loss function and a defect segmentation loss function module. Among them, the defect generation module generates the image used for synthetic training, the image repair network is responsible for repairing the defect area, and the defect prediction module inputs the image before and after repair, and calculates the area where the defect is located. The loss function is used for supervised training of the network.
[0018] Such as figure 1 As shown, this embodiment involves the semi-supervised defect detection method based on positive sample learning of the above-mentioned system, including the defect recovery process and the difference region detection process, and the difference region is obtained by restoring the image containing the defect and then c...
PUM
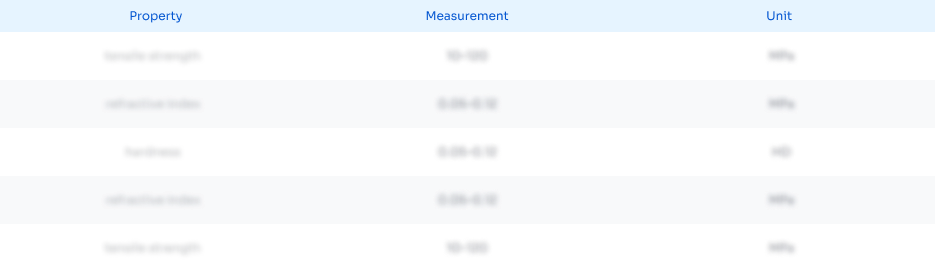
Abstract
Description
Claims
Application Information
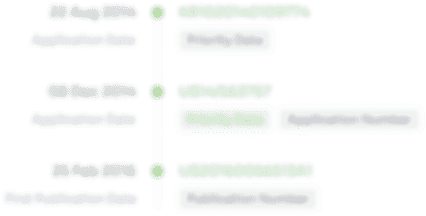
- R&D Engineer
- R&D Manager
- IP Professional
- Industry Leading Data Capabilities
- Powerful AI technology
- Patent DNA Extraction
Browse by: Latest US Patents, China's latest patents, Technical Efficacy Thesaurus, Application Domain, Technology Topic, Popular Technical Reports.
© 2024 PatSnap. All rights reserved.Legal|Privacy policy|Modern Slavery Act Transparency Statement|Sitemap|About US| Contact US: help@patsnap.com