Cold start recommendation method and device based on user interest migration and storage equipment
A technology of user interest and recommendation method, which is applied in the fields of user cold start recommendation, device and storage device, and can solve the problems of enhanced accuracy and inability to accurately predict user interest, etc.
- Summary
- Abstract
- Description
- Claims
- Application Information
AI Technical Summary
Problems solved by technology
Method used
Image
Examples
Embodiment 1
[0071] Step S21 is based on the user-topic probability distribution as the user characteristic feature, including the following steps:
[0072] Step S211. Utilize the user access log to construct a user-term matrix, including:
[0073] Step S2111. Eliminate noise data in the log data, including content without tag information and log data with too little user behavior. Among them, the content without label information means that the corresponding label cannot be matched in the content label library. In order to reduce the calculation cost, it is directly filtered; cause some disturbance. Generally, a threshold (such as 10, 20, etc.) can be determined according to the distribution of user access times, and user logs that do not meet the threshold can be directly filtered. Thus, for each user u, the user behavior vector [a 1 ,a 2 …a s ], where a i Represents content.
[0074] Step S2112. According to the content-tag dictionary, map the content in the user history browsing...
Embodiment 2
[0082] Embodiment 2: In a situation similar to Embodiment 1, the embedding method is used as the user's feature vector. Unlike the LDA model, this method only needs to use the user's access footprint. The ID feature of the content is mapped to another low-dimensional space through item2vec, and the vectorized expression of the content is obtained. Then, according to the user's recent access content records, the user's vectorized expression is obtained through weighted average. The main steps are as follows: Divide the user's access content list into multiple session fragments. Specifically, the list of user behaviors is sorted by time, and the time interval between two previous behaviors is calculated. If the interval is greater than a certain threshold (for example, 30s, 60s, etc.), segmentation is performed. At this point, the user's behavior sequence is converted into a conversation sequence. Based on the conversation sequence, the word2vec training method skip-gram is us...
PUM
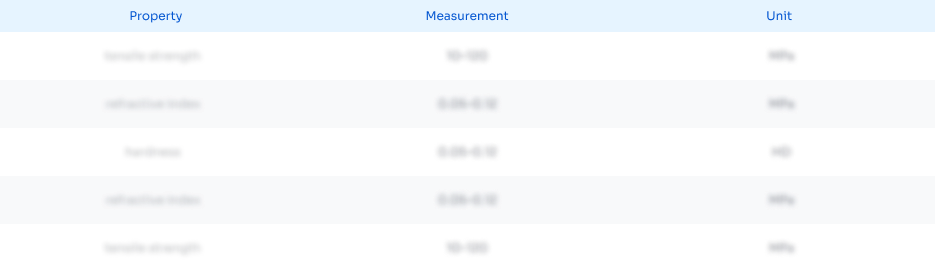
Abstract
Description
Claims
Application Information
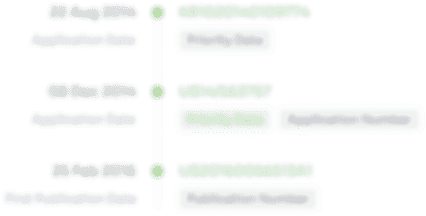
- R&D Engineer
- R&D Manager
- IP Professional
- Industry Leading Data Capabilities
- Powerful AI technology
- Patent DNA Extraction
Browse by: Latest US Patents, China's latest patents, Technical Efficacy Thesaurus, Application Domain, Technology Topic, Popular Technical Reports.
© 2024 PatSnap. All rights reserved.Legal|Privacy policy|Modern Slavery Act Transparency Statement|Sitemap|About US| Contact US: help@patsnap.com