Active learning self-iteration image classification method and system
A technology of active learning and classification methods, applied in the field of deep learning, can solve problems such as a large number of labeling costs and poor effects
- Summary
- Abstract
- Description
- Claims
- Application Information
AI Technical Summary
Problems solved by technology
Method used
Image
Examples
Embodiment Construction
[0024] In order to make the purpose, technical solutions and advantages of the present application clearer, the present application will be described and illustrated below in conjunction with the accompanying drawings and embodiments. It should be understood that the specific embodiments described here are only used to explain the present application, and are not intended to limit the present application. Based on the embodiments provided in the present application, all other embodiments obtained by persons of ordinary skill in the art without creative efforts shall fall within the protection scope of the present application.
[0025] Obviously, the accompanying drawings in the following description are only some examples or embodiments of the present application, and those skilled in the art can also apply the present application to other similar scenarios. In addition, it can also be understood that although such development efforts may be complex and lengthy, for those of ...
PUM
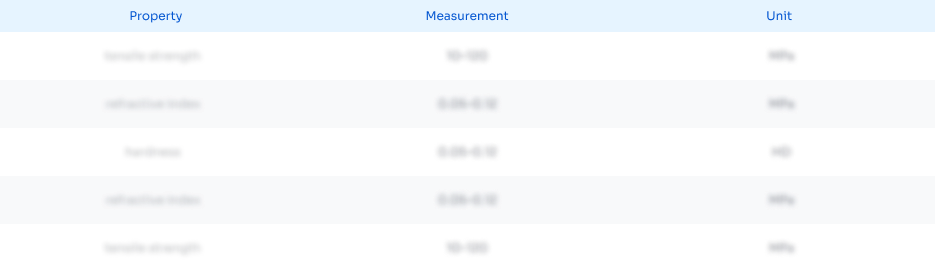
Abstract
Description
Claims
Application Information
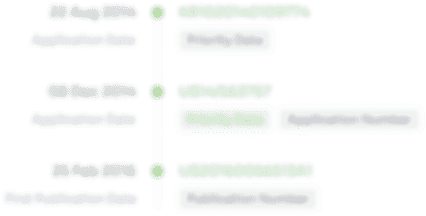
- R&D Engineer
- R&D Manager
- IP Professional
- Industry Leading Data Capabilities
- Powerful AI technology
- Patent DNA Extraction
Browse by: Latest US Patents, China's latest patents, Technical Efficacy Thesaurus, Application Domain, Technology Topic, Popular Technical Reports.
© 2024 PatSnap. All rights reserved.Legal|Privacy policy|Modern Slavery Act Transparency Statement|Sitemap|About US| Contact US: help@patsnap.com