Two-stage-based time sequence prediction method and prediction system, terminal and medium
A technology of time series and forecasting methods, applied in forecasting, neural learning methods, instruments, etc., can solve problems such as weak useful information, model accuracy does not increase but decreases, and achieves the effect of improving accuracy and realizing prediction accuracy
- Summary
- Abstract
- Description
- Claims
- Application Information
AI Technical Summary
Problems solved by technology
Method used
Image
Examples
Embodiment 1
[0058] The object of the present invention is to use the forecasting method of two-stage CEEMDAN-PSO-ELM to realize the promotion of forecasting accuracy on the basis of traditional forecasting model, concrete steps are as follows:
[0059] Step 1: Use the CEEMDAN method to decompose the original sequence to get the IMF 1 ,IMF 2 ,...,IMF n and Res;
[0060] Step 2: Put the IMF 1 ,IMF 2 ,...,IMF n Normalize with Res:
[0061]
[0062] Where: x max and x min are the maximum and minimum values in the input data respectively; y is the normalized input value.
[0063] Step 3: Bring each normalized subsequence into the PSO-ELM model for prediction, denormalize the output result, and obtain the prediction result Y 1 ,Y 2 ,...Y n ,Y n+1 ;
[0064] Step 4: Set Y 1 ,Y 2 ,...Y n ,Y n+1 Sum up to get the first stage prediction result Y sum , subtract the actual value from Y sum Get the error sequence E.
[0065] Step 5: Use the CEEMDAN method to decompose the erro...
Embodiment 2
[0071] The embodiment of the present invention adopts the monthly mean value data of sunspots, which are derived from the official website (http: / / sidc.oma.be / silso / datafiles) of the Belgian Royal Astronomical Observatory solar action data analysis center (Solar Influence Data Analysis Center, SIDC) ), its prediction process is as follows figure 2 shown. The first stage: use CEEMDAN to stabilize the sunspot number series of monthly mean value as image 3 shown; ELM models were established for each sub-sequence after decomposition, and the ELM parameters of each sub-model were optimized with the PSO algorithm, as shown in Table 1 and Figure 4 As shown, the prediction results of each component are superimposed to obtain the first-stage prediction results. The second stage: CEEMDAN-PSO-ELM modeling is performed on the residual obtained in the first stage, and the intermediate process is as follows Figure 5 , Table 2 and Figure 6 As shown, the prediction results of the sec...
Embodiment 3
[0083] CEEMDAN decomposition principle
[0084] Huang proposed an Empirical Mode Decomposition (EMD) method that can decompose any signal into Intrinsic Mode Functions (IMF). M.A.Colorminas proposed the CEEMDAN decomposition method based on the research of Huang et al. CEEMDAN utilizes the characteristics of zero-mean Gaussian white noise to make the decomposition effect of signal data more complete. The specific processing process is as follows:
[0085] Step 1: Add standard normal distribution white noise w of different amplitudes to the given target signal x(n) i (n), construct the signal sequence of the i-th experiment as
[0086] x i (n)=x(n)+γw i (n) (i=1,...,I) (1)
[0087] Step 2: In the first stage, use the EMD method to decompose the target signal, obtain the first modal component and take the mean value
[0088]
[0089] The margin signal of the first stage is expressed as:
[0090]
[0091] Step 3: Define E k (·) is the kth IMF component after performi...
PUM
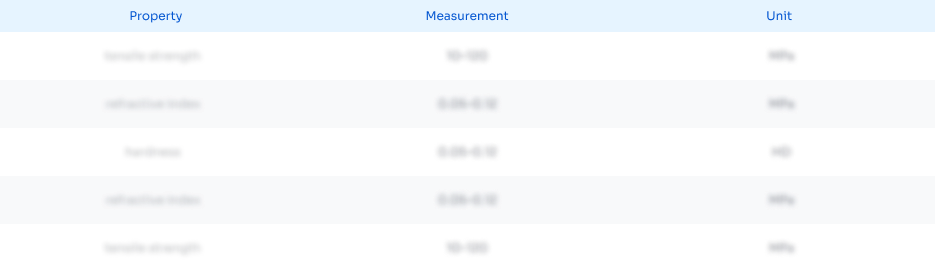
Abstract
Description
Claims
Application Information
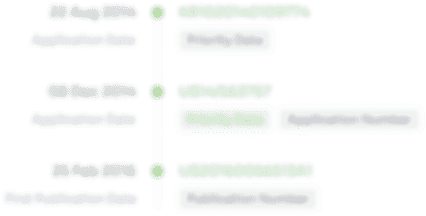
- R&D Engineer
- R&D Manager
- IP Professional
- Industry Leading Data Capabilities
- Powerful AI technology
- Patent DNA Extraction
Browse by: Latest US Patents, China's latest patents, Technical Efficacy Thesaurus, Application Domain, Technology Topic, Popular Technical Reports.
© 2024 PatSnap. All rights reserved.Legal|Privacy policy|Modern Slavery Act Transparency Statement|Sitemap|About US| Contact US: help@patsnap.com