Low-illumination image enhancement method based on improved depth separable generative adversarial network
An image enhancement, low illumination technology, applied in image enhancement, biological neural network model, image analysis and other directions, can solve the problems of weak robustness, insufficient memory, high computational complexity and time consumption, etc. The effect of complexity, reducing the amount of model parameters, and increasing computational efficiency
- Summary
- Abstract
- Description
- Claims
- Application Information
AI Technical Summary
Problems solved by technology
Method used
Image
Examples
Embodiment
[0084] (1) Generate confrontation network
[0085] The new framework for estimating a generative model via an adversarial process trains two models simultaneously: a generative model G that captures the distribution of the data, and a discriminative model D that estimates the probability that a sample came from the training data.
[0086] The generated confrontation network is composed of two parts: the generative model G (generative model) and the discriminative model D (discriminative model). Generative models are used to learn the distribution of real data. A discriminative model is a binary classifier that distinguishes whether the input is real data or generated data. X is real data, conforming to P r (x) distribution. Z is a latent space variable, conforming to P z(z) distribution, such as Gaussian distribution or uniform distribution. Then sample from the hypothetical hidden space z, and generate data x'=G(z) after generating the model G. Then the real data and th...
PUM
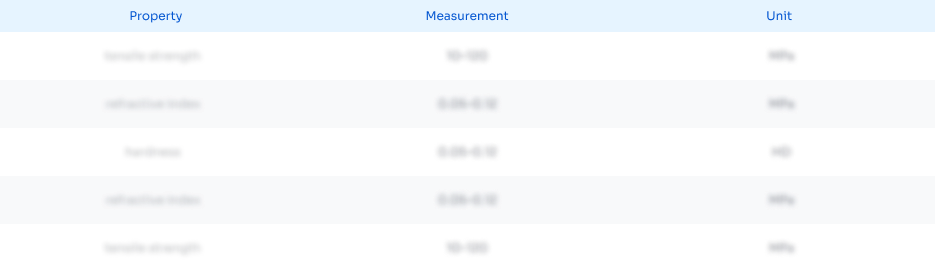
Abstract
Description
Claims
Application Information
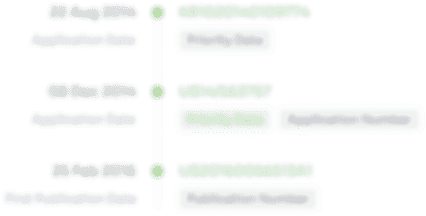
- R&D Engineer
- R&D Manager
- IP Professional
- Industry Leading Data Capabilities
- Powerful AI technology
- Patent DNA Extraction
Browse by: Latest US Patents, China's latest patents, Technical Efficacy Thesaurus, Application Domain, Technology Topic, Popular Technical Reports.
© 2024 PatSnap. All rights reserved.Legal|Privacy policy|Modern Slavery Act Transparency Statement|Sitemap|About US| Contact US: help@patsnap.com