A method and system for automatic driving decision-making control based on layered reinforcement learning
A reinforcement learning and automatic driving technology, applied in machine learning, control devices, instruments, etc., can solve problems that cannot meet the decision-making and control requirements of automatic driving, and achieve training that is easy to complete, training tasks are clear, and rapid acceleration and deceleration can be avoided Effect
- Summary
- Abstract
- Description
- Claims
- Application Information
AI Technical Summary
Problems solved by technology
Method used
Image
Examples
Embodiment Construction
[0039] The present invention will be further described below with reference to the accompanying drawings, but the protection scope of the present invention is not limited thereto.
[0040] figure 1 It is a flowchart of an automatic driving decision-making control method based on layered reinforcement learning, and the method of the present invention includes an action layer Agent and a decision-making layer Agent, as follows:
[0041] Action layer Agent obtains road environment information through environment interaction The action layer Agent obtains the state quantity After that, a definite action μ is obtained t , and then determine the action μ by giving the t an exploration noise n t , to synthesize an exploratory action a t . The smart car is performing an action a t Feedback from the environment and r l . Feedback amount is the new state quantity, r l to perform action a t rewards received afterwards. By changing the task, the action layer Agent learns...
PUM
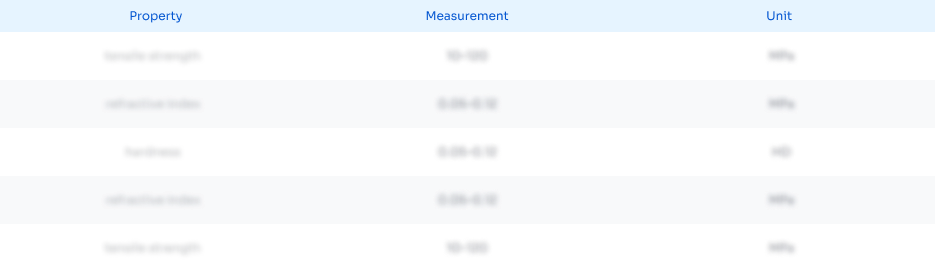
Abstract
Description
Claims
Application Information
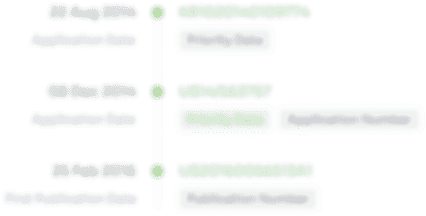
- R&D Engineer
- R&D Manager
- IP Professional
- Industry Leading Data Capabilities
- Powerful AI technology
- Patent DNA Extraction
Browse by: Latest US Patents, China's latest patents, Technical Efficacy Thesaurus, Application Domain, Technology Topic, Popular Technical Reports.
© 2024 PatSnap. All rights reserved.Legal|Privacy policy|Modern Slavery Act Transparency Statement|Sitemap|About US| Contact US: help@patsnap.com