Subway inspection AGV fault detection method
A fault detection and subway technology, applied in neural learning methods, inspection time patrols, biological neural network models, etc., can solve problems such as high cost of manual subway fault detection vehicles, hidden dangers to personal safety, and inability to meet complex environmental detection, etc., to achieve The effect of improving recognition accuracy and recognition speed
- Summary
- Abstract
- Description
- Claims
- Application Information
AI Technical Summary
Problems solved by technology
Method used
Image
Examples
Embodiment Construction
[0047] In order to make the technical means, creative features, goals and effects achieved by the present invention easy to understand, the present invention will be further elaborated below in conjunction with illustrations and specific embodiments.
[0048] The subway inspection AGV fault detection method that the present invention proposes comprises:
[0049] 1) if figure 1 , establish the first deep neural network to identify the camera information collected by the subway inspection AGV, extract the complete information of the subway, shield other image noise, and establish the corresponding activation function and loss function; the first deep neural network contains 26 volumes Multilayer, 2 fully connected layers and a double-layer neural network. After the image captured by the camera of the inspection robot is segmented, it is input into the first layer of deep neural network, and the image is deeply processed through the convolution layer and the maximum pooling layer...
PUM
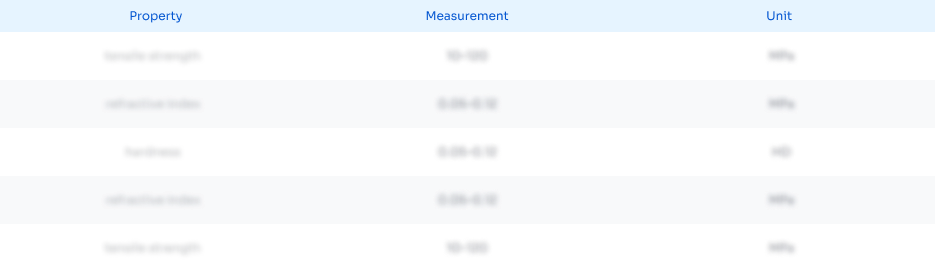
Abstract
Description
Claims
Application Information
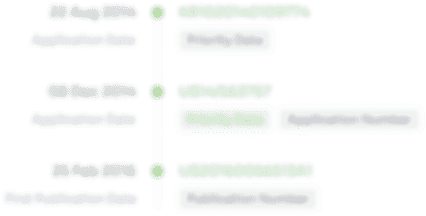
- R&D Engineer
- R&D Manager
- IP Professional
- Industry Leading Data Capabilities
- Powerful AI technology
- Patent DNA Extraction
Browse by: Latest US Patents, China's latest patents, Technical Efficacy Thesaurus, Application Domain, Technology Topic, Popular Technical Reports.
© 2024 PatSnap. All rights reserved.Legal|Privacy policy|Modern Slavery Act Transparency Statement|Sitemap|About US| Contact US: help@patsnap.com