Short video automatic labeling method based on feature and multi-label enhanced representation
An automatic labeling and short video technology, applied in the field of short video, can solve problems such as rising labor costs, low efficiency, and complicated processes, and achieve the effect of improving accuracy
- Summary
- Abstract
- Description
- Claims
- Application Information
AI Technical Summary
Problems solved by technology
Method used
Image
Examples
Embodiment 1
[0029] The embodiment of the present invention provides a short video automatic labeling method based on feature and multi-label enhanced representation, see figure 1 , the method includes the following steps:
[0030] 101: Reconstruct the original feature matrix by using the dictionary mapping matrix, the product of the public low-rank representation and the sparse error matrix, so as to form a multi-view low-rank representation item as a whole (the first objective function);
[0031] 102: By clustering the overall data set, obtain all data sets and potential label correlation information in different clusters, and form a global and local label correlation learning item (second objective function);
[0032] 103: Use the public low-rank representation as the predicted label, subtract it from the real label to obtain the labeling error and minimize it, forming a minimum labeling error term (the third objective function);
[0033] 104: Obtain the total objective function weight...
Embodiment 2
[0039] The scheme in embodiment 1 is further introduced below in conjunction with calculation formula and examples, see the following description for details:
[0040] 201: Use the penultimate fully connected layer of the VGG-m-2048 network to extract 2048-dimensional advanced visual semantic features, use the TDD model and Fisher Vector to extract 2048-dimensional trajectory features from the video dataset, and use the l2 norm for each The characteristics of the perspective are standardized to obtain the final standardized multi-view feature X 1 and x 2 .
[0041] 202: Determine the characteristics X of different viewing angles i The public low-rank representation of L, according to the formula:
[0042] x i =D i L+E i ,i=1,2,...,V
[0043] (1)
[0044] Among them, using the dictionary mapping matrix D i feature X from different perspectives i Mapping to the public low-rank representation L, and making the rank of L reach the lowest, the rank of the matrix can be re...
PUM
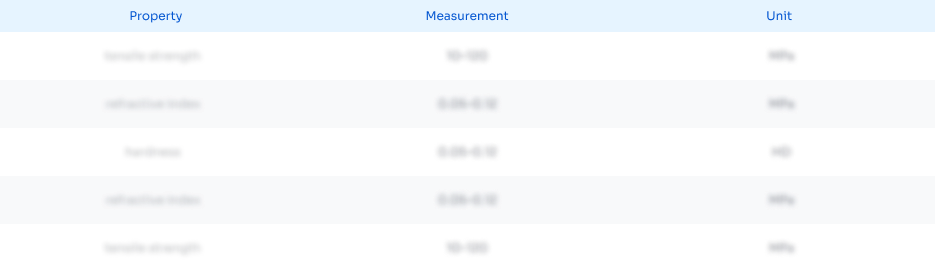
Abstract
Description
Claims
Application Information
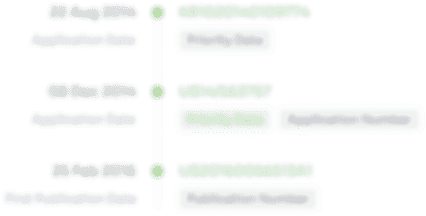
- Generate Ideas
- Intellectual Property
- Life Sciences
- Materials
- Tech Scout
- Unparalleled Data Quality
- Higher Quality Content
- 60% Fewer Hallucinations
Browse by: Latest US Patents, China's latest patents, Technical Efficacy Thesaurus, Application Domain, Technology Topic, Popular Technical Reports.
© 2025 PatSnap. All rights reserved.Legal|Privacy policy|Modern Slavery Act Transparency Statement|Sitemap|About US| Contact US: help@patsnap.com