Block pedestrian flow prediction method and system based on space-time diagram convolutional neural network
A technology of convolutional neural network and prediction method, which is applied in the field of block flow prediction based on spatio-temporal graph convolutional neural network, can solve the problems that cannot take into account both time and space influencing factors at the same time, do not meet actual needs, and cannot adapt to complex flow prediction schemes. problems such as the dynamic mode of the traffic flow, to achieve the effect of good flow prediction performance
- Summary
- Abstract
- Description
- Claims
- Application Information
AI Technical Summary
Problems solved by technology
Method used
Image
Examples
specific Embodiment approach
[0090] In order to train the model, the system needs to collect large-scale moving trajectories to generate human flow and construct a space-time dynamic map. If large-scale fine-grained trajectories are difficult to collect, this step can also be directly replaced by aggregated human flow. In addition, the PoI data in the city should also be collected and classified to obtain the characteristic distribution of PoI. Then, the city needs to be divided according to the characteristic distribution of PoI, and the specific implementation method is to apply the clustering algorithm. further as figure 2 As shown in the top flow, the neighborhood of each class center is retained as the ground truth for the calculation of the second part of the loss function. Next, segment the flow data sequence by time. For example, the data of 30 days can be trained in the first 24 days, and the parameters of the model can be adjusted in the last 6 days. The model is trained according to the metho...
PUM
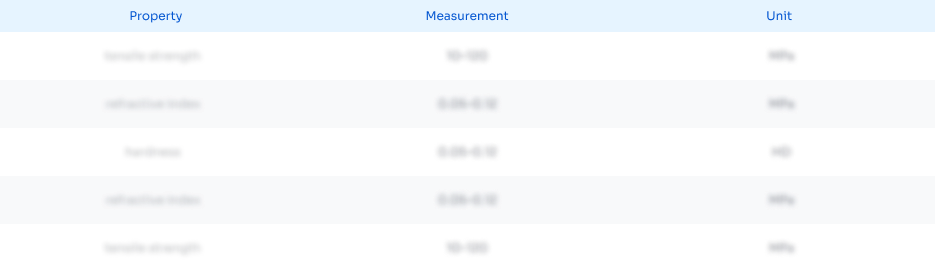
Abstract
Description
Claims
Application Information
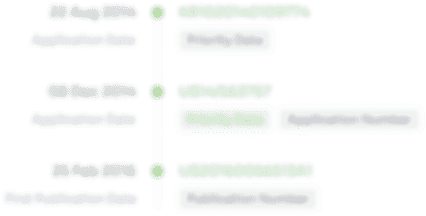
- R&D Engineer
- R&D Manager
- IP Professional
- Industry Leading Data Capabilities
- Powerful AI technology
- Patent DNA Extraction
Browse by: Latest US Patents, China's latest patents, Technical Efficacy Thesaurus, Application Domain, Technology Topic, Popular Technical Reports.
© 2024 PatSnap. All rights reserved.Legal|Privacy policy|Modern Slavery Act Transparency Statement|Sitemap|About US| Contact US: help@patsnap.com