Uniform linear array low sidelobe beam forming optimization method under multiple constraints
A uniform linear array and optimization method technology, applied in radio wave measurement systems, radio wave reflection/reradiation, instruments, etc., can solve the problems of slow algorithm convergence, non-convergence, and failure to consider the requirements of array gain, etc., to achieve The array gain is large, the convergence speed is fast, and the convergence effect is good
- Summary
- Abstract
- Description
- Claims
- Application Information
AI Technical Summary
Problems solved by technology
Method used
Image
Examples
Embodiment 1
[0122] Embodiment 1: The number of uniform linear array elements is 16, the element interval is half a wavelength, the beam points to 0°, and the upper and lower bounds of the main lobe level are P l = 21.78dB and P u =21.98dB, the upper limit level of the side lobe level is η=0.083dB, and the lower limit of the weight amplitude range is σ=1 / 2 7 , the maximum number of iterations is K max =10000, the maximum tolerance is ε y =ε m =10 -5 , the initial value of the penalty factor is ρ y = ρ m =10 -4 , the value of the dual auxiliary variable does not exceed ν=500000, δ 1 =0.99, δ 2 =1.02, other parameters are initialized randomly between [0,1]. Considering that the noise covariance matrix is R=I, the maximum array gain is 12.0411dB without other constraints. Compare the optimization effect of the ADPM algorithm that the present invention adopts and alternate direction multiplier (ADMM) algorithm, penalty factor is fixed as ρ y = ρ m =10 -4 , other conditions remai...
Embodiment 2
[0124] Embodiment 2: In order to analyze the performance advantages of the method of the present invention, different initial values of penalty factors are set, and based on the above parameters, the convergence speeds of the two algorithms are compared.
[0125] Figure 7 is the penalty factor ρ y respectively set to ρ y =0.0001, ρ y = 0.001 and ρ y =0.01, the residual Δr corresponding to the two algorithms y the convergence curve; Figure 8 is the penalty factor ρ m respectively set to ρ m =0.0001, ρ m = 0.001 and ρ m =0.01, the residual Δr corresponding to the two algorithms m the convergence curve. from Figure 7 with Figure 8 It can be seen from the figure that setting different initial values of the penalty factor has little influence on the convergence speed performance of the ADPM algorithm, and the convergence speed of the ADPM algorithm is faster when the same initial value of the penalty factor is set.
[0126] In summary, the multi-constrained uni...
PUM
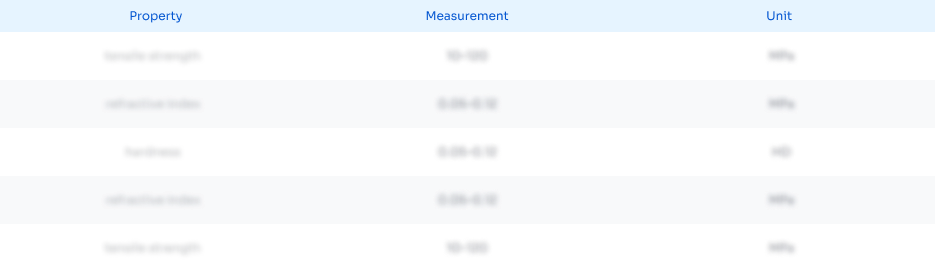
Abstract
Description
Claims
Application Information
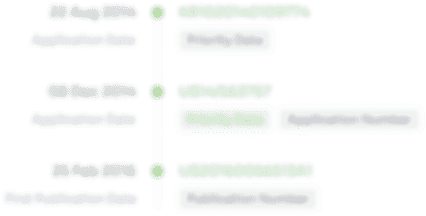
- R&D Engineer
- R&D Manager
- IP Professional
- Industry Leading Data Capabilities
- Powerful AI technology
- Patent DNA Extraction
Browse by: Latest US Patents, China's latest patents, Technical Efficacy Thesaurus, Application Domain, Technology Topic, Popular Technical Reports.
© 2024 PatSnap. All rights reserved.Legal|Privacy policy|Modern Slavery Act Transparency Statement|Sitemap|About US| Contact US: help@patsnap.com