Key opinion consumer mining method, device and equipment and medium
A technology of consumers and opinions, applied in business, instruments, data processing applications, etc., can solve the problem of difficulty in ensuring the efficiency and accuracy of identification
- Summary
- Abstract
- Description
- Claims
- Application Information
AI Technical Summary
Problems solved by technology
Method used
Image
Examples
Embodiment 1
[0047] Embodiment 1 of the present invention provides a key opinion consumer mining method, figure 1 A flow chart showing Embodiment 1 of the method for mining key opinion consumers of the present invention, including: a method for mining key opinion consumers, characterized in that it includes:
[0048] Step S101: Determine a first preset number of target features in the activity field, community field, and consumption field according to the determined key opinion consumer KOC and the determined non-KOC, as well as the feature values corresponding to each of the target features and target sample space;
[0049]Determine the target characteristics in the activity field, community field and consumption field according to the currently identified key consumer KOC and identified non-KOC, and the identified KOC and identified non-KOC can be extracted from the database of identified data Obtained, for example, extracting 3000 determined KOCs and 3000 determined non-KOCs, as a sp...
specific Embodiment approach
[0050] As a specific implementation, the method of defining the characteristic value of the target characteristic can be as follows:
[0051] In the field of activity dimension:
[0052] Participation frequency of publicity activities: In daily operation, the monthly granularity is the number of times each user participates in publicity activities. More than or equal to 4 times is 1 point, less than 4 times and greater than or equal to 2 times is 0.5 points, less than 2 times is 0 Minute;
[0053] Coupon activity participation frequency: with monthly granularity, the number of coupons received by each user per month accounts for the percentage of monthly card coupon activities, 1 point for more than or equal to 60% of times, 0.5 points for less than 60% and greater than or equal to 40%, 0.5 points for less than 60% 40% is 0 points;
[0054] Activity sharing frequency: 1 point for each user sharing 80% to 50% of activities per month, 0.5 points for sharing 50% to 20% of activ...
Embodiment approach
[0082] A specific implementation, the accuracy of the labeling results can be evaluated and calculated according to the ROC receiver operating characteristic curve, the area under the ROC curve is called the AUC (Area Under Curve) value, which means randomly selecting a positive sample and a negative sample, The probability that the current classification algorithm ranks the positive sample score before the negative sample. The larger the AUC, the more accurate the classification result.
[0083] AUC is calculated based on the two values of true positive rate TPR and false positive rate FPR, true positive rate TPR=TP / (TP+FN), false positive rate FPR=FP / (TN+FP);
[0084] Among them, TP: true example, FP: false positive example, TN: true negative example, FN: false negative example, as shown in the following table:
[0085]
[0086] TP true cases are the number of samples where the real situation is positive and the classification result is positive; FP false positives are ...
PUM
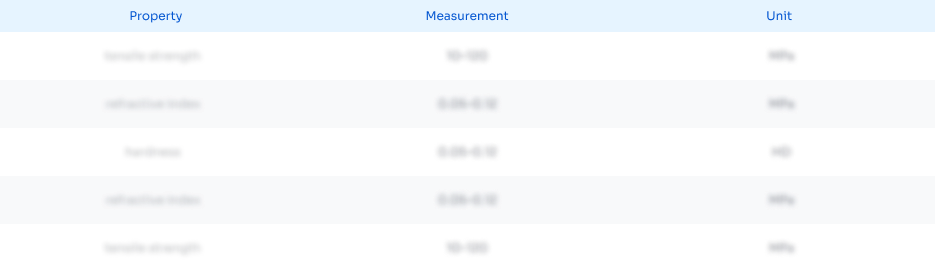
Abstract
Description
Claims
Application Information
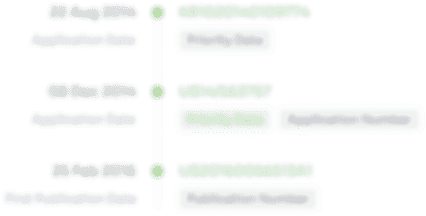
- R&D Engineer
- R&D Manager
- IP Professional
- Industry Leading Data Capabilities
- Powerful AI technology
- Patent DNA Extraction
Browse by: Latest US Patents, China's latest patents, Technical Efficacy Thesaurus, Application Domain, Technology Topic, Popular Technical Reports.
© 2024 PatSnap. All rights reserved.Legal|Privacy policy|Modern Slavery Act Transparency Statement|Sitemap|About US| Contact US: help@patsnap.com